Modal distance constraint-based multimodal fusion image classification method
A technique for fusing images and distance constraints, applied in the field of image processing, can solve problems such as the need to improve the classification accuracy and not consider the relationship between different modal data
- Summary
- Abstract
- Description
- Claims
- Application Information
AI Technical Summary
Problems solved by technology
Method used
Image
Examples
Embodiment 1
[0053] The invention discloses a multimodal fusion image classification method based on modal distance constraints, such as figure 1 As shown, the method includes the steps of:
[0054] The first step, obtaining data, specifically: obtaining rs-fMRI data and DTI data of a plurality of subjects, and performing preprocessing, obtaining preprocessed rs-fMRI data and preprocessed DTI data;
[0055] The second step is to construct the feature vector of the brain function network and the feature vector of the brain structure network. The details are as follows:
[0056] The construction of the brain function network feature vector is based on the preprocessed rs-fMRI data, specifically: using the automatic anatomy label template to generate 90 cortical and subcutaneous nuclei regions, and removing the cerebellum; The Pearson correlation coefficient of the region to the average time series; the nodes in the brain function network are defined as ninety cortical and subcutaneous nucle...
PUM
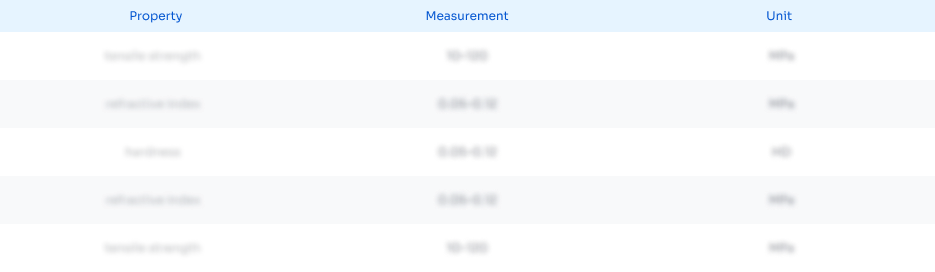
Abstract
Description
Claims
Application Information
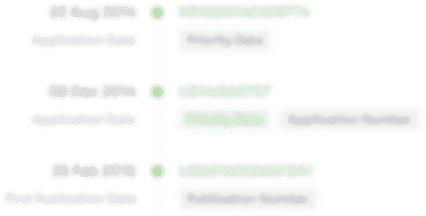
- R&D Engineer
- R&D Manager
- IP Professional
- Industry Leading Data Capabilities
- Powerful AI technology
- Patent DNA Extraction
Browse by: Latest US Patents, China's latest patents, Technical Efficacy Thesaurus, Application Domain, Technology Topic.
© 2024 PatSnap. All rights reserved.Legal|Privacy policy|Modern Slavery Act Transparency Statement|Sitemap