Least squares support vector machine soft measurement method based on distributed parallel local optimization parameters
A technology of support vector machine and local optimization, applied in the direction of specific mathematical model, design optimization/simulation, genetic model, etc., it can solve the problem of slow calculation speed of LSSVM algorithm, and achieve the effect of reducing calculation cost
- Summary
- Abstract
- Description
- Claims
- Application Information
AI Technical Summary
Problems solved by technology
Method used
Image
Examples
Embodiment Construction
[0046] The present invention will be described in detail below according to the accompanying drawings and preferred embodiments, and the purpose and effect of the present invention will become clearer. The present invention will be further described in detail below in conjunction with the accompanying drawings and embodiments. It should be understood that the specific embodiments described here are only used to explain the present invention, not to limit the present invention.
[0047] A least squares support vector machine soft sensor method based on distributed parallel local optimization parameters, characterized in that the modeling process of the least squares support vector machine based on local optimization parameters is as follows:
[0048] (a) normalize the training sample set and the test sample set;
[0049] (b) Find a training sample with the closest Euclidean distance to each normalized test sample in the normalized training sample set, and combine these found tr...
PUM
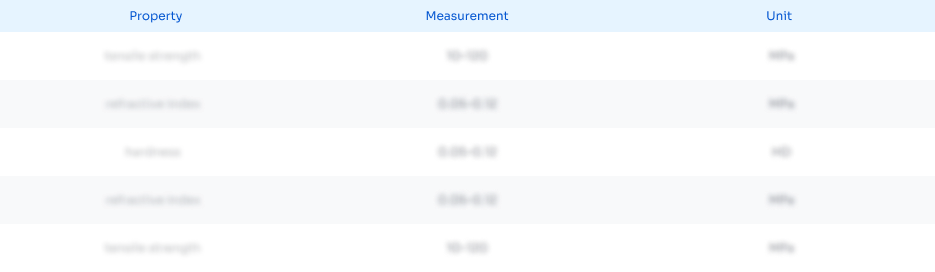
Abstract
Description
Claims
Application Information
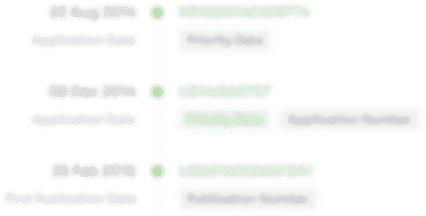
- Generate Ideas
- Intellectual Property
- Life Sciences
- Materials
- Tech Scout
- Unparalleled Data Quality
- Higher Quality Content
- 60% Fewer Hallucinations
Browse by: Latest US Patents, China's latest patents, Technical Efficacy Thesaurus, Application Domain, Technology Topic, Popular Technical Reports.
© 2025 PatSnap. All rights reserved.Legal|Privacy policy|Modern Slavery Act Transparency Statement|Sitemap|About US| Contact US: help@patsnap.com