Plug-in hybrid electric vehicle energy management method based on deep reinforcement learning
A hybrid vehicle and reinforcement learning technology, applied in data processing applications, biological neural network models, instruments, etc., can solve problems such as increased computation, continuity, discreteness, randomness, and dimension catastrophe
- Summary
- Abstract
- Description
- Claims
- Application Information
AI Technical Summary
Problems solved by technology
Method used
Image
Examples
Embodiment Construction
[0071] The technical solution of the present application will be further explained in detail below in conjunction with the accompanying drawings.
[0072] like figure 1 As shown, the present invention provides a plug-in hybrid electric vehicle energy management method, specifically comprising the following steps:
[0073] Step 1. Use the deep convolutional neural network and the long-short-term memory neural network to perform representation extraction on the vehicle visual information and traffic state information respectively.
[0074] Step 2. Carry out dimensionality reduction and fusion processing on the vehicle-mounted visual information and traffic state information extracted in step 1, as well as the vehicle's own state information, slope information and other working condition representations, to obtain a low-dimensional continuous working condition state .
[0075] Step 3, using the low-dimensional continuous working condition state obtained in the step 2 as an inpu...
PUM
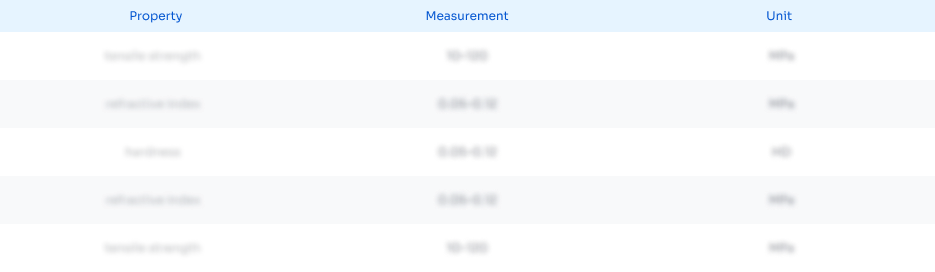
Abstract
Description
Claims
Application Information
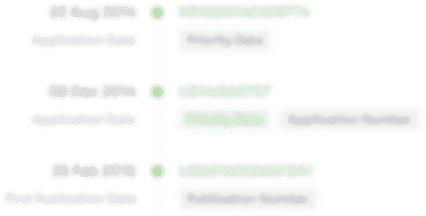
- R&D Engineer
- R&D Manager
- IP Professional
- Industry Leading Data Capabilities
- Powerful AI technology
- Patent DNA Extraction
Browse by: Latest US Patents, China's latest patents, Technical Efficacy Thesaurus, Application Domain, Technology Topic, Popular Technical Reports.
© 2024 PatSnap. All rights reserved.Legal|Privacy policy|Modern Slavery Act Transparency Statement|Sitemap|About US| Contact US: help@patsnap.com