Pedestrian detection method based on semantic segmentation information
A technology of semantic segmentation and pedestrian detection, which is applied in the field of pedestrian detection based on neural network, can solve the problems of blurred semantic information segmentation, difficulty in distinguishing pedestrians from the background, and low detection accuracy, so as to improve positioning accuracy, improve performance, and avoid The effect of false detection and missed detection
- Summary
- Abstract
- Description
- Claims
- Application Information
AI Technical Summary
Problems solved by technology
Method used
Image
Examples
Embodiment 1
[0043] A pedestrian detection method based on semantic segmentation information, comprising the following steps
[0044] Step 1: Input the original RGB image in the training set sample into the backbone network in the overall network, input the corresponding semantic segmentation image into the branch network in the overall network, and set the loss function of the overall network to complete the training;
[0045] Step 2: Input the original RGB image in the test set sample into the backbone network in the overall network that has been trained to perform convolutional feature extraction to generate a multi-layer feature map;
[0046] Step 3: Input the multi-layer feature map into the area generation network in the trained overall network to extract the pedestrian candidate frame and generate the pedestrian candidate area;
[0047] Step 4: After the classification and regression network in the trained overall network classifies and locates the pedestrian candidate area, it outp...
Embodiment 2
[0049] Step 1 includes the following steps:
[0050] Step 1.1: Initialize the branch network of the backbone network in the overall network to determine the loss proportion λ i ;
[0051] The initialization is as follows: the parameter initialization of the backbone network adopts the pre-training initialization method, the initialization of the branch network adopts random initialization, the first 60,000 iterations, the learning rate is 0.001, and the last 20,000 iterations, the learning rate is 0.0001, the momentum is set to 0.9, and the weight The attenuation is set to 0.0005, and the loss specific gravity λ i Take 1, the loss proportion is determined according to different training sets.
[0052] Step 1.2: Input the original RGB image into the backbone network and the corresponding semantic segmentation image into the branch network to complete the selection of foreground samples and background samples and generate multi-layer feature maps;
[0053]Select the foregroun...
PUM
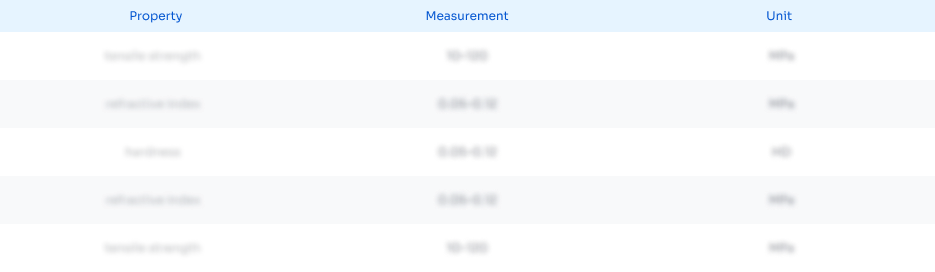
Abstract
Description
Claims
Application Information
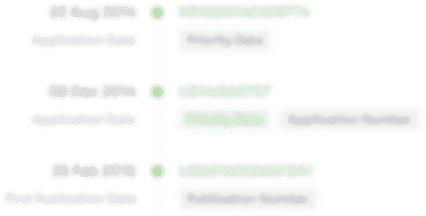
- R&D Engineer
- R&D Manager
- IP Professional
- Industry Leading Data Capabilities
- Powerful AI technology
- Patent DNA Extraction
Browse by: Latest US Patents, China's latest patents, Technical Efficacy Thesaurus, Application Domain, Technology Topic, Popular Technical Reports.
© 2024 PatSnap. All rights reserved.Legal|Privacy policy|Modern Slavery Act Transparency Statement|Sitemap|About US| Contact US: help@patsnap.com