Multi-object tracking method based on deep reinforcement learning
A multi-target tracking and reinforcement learning technology, applied in the field of multi-target tracking methods and devices based on deep reinforcement learning, can solve the problems of occlusion and noise sensitivity, inaccurate labeling, false detection, etc., and achieve the effect of overcoming occlusion and improving performance
- Summary
- Abstract
- Description
- Claims
- Application Information
AI Technical Summary
Problems solved by technology
Method used
Image
Examples
Embodiment Construction
[0034]Embodiments of the present invention are described in detail below, examples of which are shown in the drawings, wherein the same or similar reference numerals designate the same or similar elements or elements having the same or similar functions throughout. The embodiments described below by referring to the figures are exemplary and are intended to explain the present invention and should not be construed as limiting the present invention.
[0035] The following describes the multi-target tracking method and device based on deep enhanced learning according to the embodiments of the present invention with reference to the accompanying drawings. First, the multi-target tracking method based on deep enhanced learning according to the embodiments of the present invention will be described with reference to the accompanying drawings.
[0036] figure 1 It is a flowchart of a multi-target tracking method based on deep reinforcement learning according to an embodiment of the ...
PUM
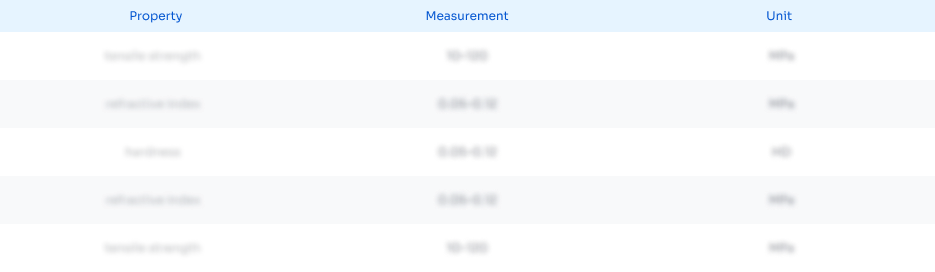
Abstract
Description
Claims
Application Information
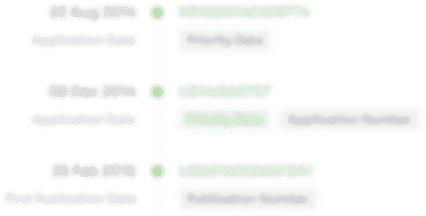
- R&D
- Intellectual Property
- Life Sciences
- Materials
- Tech Scout
- Unparalleled Data Quality
- Higher Quality Content
- 60% Fewer Hallucinations
Browse by: Latest US Patents, China's latest patents, Technical Efficacy Thesaurus, Application Domain, Technology Topic, Popular Technical Reports.
© 2025 PatSnap. All rights reserved.Legal|Privacy policy|Modern Slavery Act Transparency Statement|Sitemap|About US| Contact US: help@patsnap.com