Multi-freedom-degree synchronous electromyographic control method based on deep learning
A technology of deep learning and degrees of freedom, applied in the field of myoelectric control, can solve the problems of lack of information of myoelectric signals, lack of interrelationship of degrees of freedom, etc., and achieve the effect of strong adaptability, good movement direction and movement trend
- Summary
- Abstract
- Description
- Claims
- Application Information
AI Technical Summary
Problems solved by technology
Method used
Image
Examples
specific Embodiment approach 1
[0020] Specific implementation mode one: as figure 1 with 2 As shown, the multi-degree-of-freedom synchronous myoelectric control method based on deep learning described in this embodiment is specifically carried out in accordance with the following steps:
[0021] Step 1. Synchronous acquisition and storage of original EMG signals and corresponding motion information;
[0022] Step 2. Establish an initial multi-degree-of-freedom synchronous myoelectric control model based on a one-dimensional convolutional neural network;
[0023] Step 3. Input the original EMG signals of each group of experimental data collected in Step 1 into the initial multi-degree-of-freedom synchronous EMG control model established in Step 2, and correspondingly take the motion information of each group of experimental data as the output target, and obtain The final multi-degree-of-freedom synchronous myoelectric control model;
[0024] Step 4, collect the subject's original myoelectric signal, input...
specific Embodiment approach 2
[0029] Specific embodiment two: the difference between this embodiment and specific embodiment one is: the specific process of synchronous collection and storage of wrist motion information and electromyographic signals in step one is:
[0030] Select no less than 9 subjects to participate in the data collection. Each arm of the subject needs to collect no less than 3 sets of EMG data, and the two arms alternately collect data; that is, after collecting a set of data , the electrode is removed and placed on the other arm for data collection; the electrode will deviate from the last placement position by no more than 1cm during the repeated wearing process;
[0031] The movement information of the 3 degrees of freedom of the wrist is converted into the movement information of the horizontal movement, vertical movement and angular rotation of the cursor projected on the screen through the cross laser transmitter; when collecting data, the operator is only required to plan two at ...
specific Embodiment approach 3
[0035] Specific implementation mode three: as figure 2 shown. The difference between this embodiment and the specific embodiment two is: the specific process of step three is:
[0036] The original EMG signal collected in step 1 is intercepted by windowing and used as input. After N convolutional layers and pooling layers, the N+1th convolutional layer is the convolutional layer. The output length after convolution in the convolutional layer is is 1, and then through the further feature extraction of the fully connected layer, the information of multiple target degrees of freedom is finally output through the linear regression layer;
[0037] Among them, the value of N is greater than or equal to 1, and there is an activation function layer between each convolutional layer and the pooling layer, and an activation function layer between the N+1th convolutional layer and the fully connected layer.
[0038] The convolutional layer in this embodiment can change the expression f...
PUM
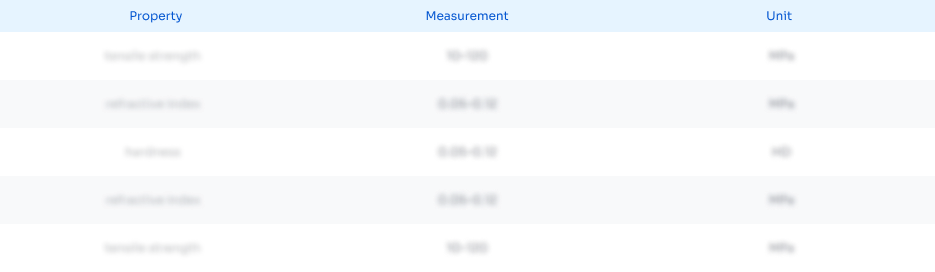
Abstract
Description
Claims
Application Information
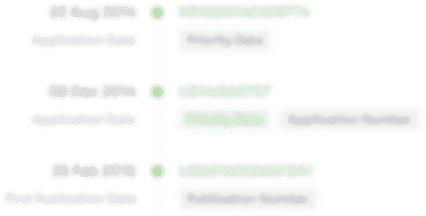
- R&D Engineer
- R&D Manager
- IP Professional
- Industry Leading Data Capabilities
- Powerful AI technology
- Patent DNA Extraction
Browse by: Latest US Patents, China's latest patents, Technical Efficacy Thesaurus, Application Domain, Technology Topic, Popular Technical Reports.
© 2024 PatSnap. All rights reserved.Legal|Privacy policy|Modern Slavery Act Transparency Statement|Sitemap|About US| Contact US: help@patsnap.com