Chinese formal text word segmentation method based on active learning
An active learning and text segmentation technology, applied in special data processing applications, instruments, electronic digital data processing and other directions, can solve a large number of manual labeling data, can not solve the boundary ambiguity and unregistered words, word meaning differences and other problems, to reduce labor The effect of labeling data
- Summary
- Abstract
- Description
- Claims
- Application Information
AI Technical Summary
Problems solved by technology
Method used
Image
Examples
Embodiment 1
[0035] The present invention provides a method of active learning, such as figure 1 shown, including the following steps:
[0036] Step 1: Use the existing small amount of labeled data to learn and train to obtain a prediction model;
[0037] Step 2: Predict the unlabeled data through the prediction model obtained through training, so as to obtain the prediction result, and the prediction result is to select the data to be labeled from the unlabeled data;
[0038] Step 3: Use the sampling method to select the most informative data fragments from the data to be labeled and submit them to experts for labeling;
[0039] Step 4: combining the labeled data and the labeled data to retrain the prediction model, and iterate continuously until a certain labeling ratio is reached to end the iteration;
[0040] Specifically, when there is little or no labeled data, manually labeling data is a time-consuming and labor-intensive task. Active learning is to use learning algorithms to sub...
experiment example
[0088] Data generally includes formal data and informal data. For example, literature and People's Daily are formal data, while Weibo is informal data. The data used in this paper come from 16 core journals such as "Computer Science", "Computer Application", "Journal of Software", "Journal of Medical Informatics", and a total of 10,000 paper titles are used. The data in this article is a formal text, and it contains a large amount of information and has the characteristics of short and concise.
[0089] Experimental evaluation
[0090] This application uses the commonly used F-score to measure the performance of the classifier, that is, the harmonic mean of the precision rate and the recall rate. Here we use the confusion matrix as shown in the table to introduce the precision and recall of the experiments in this paper.
[0091] Table 1 Mixing matrix table
[0092]
Segmentation after word segmentation
Not segmented after word segmentation
should act...
PUM
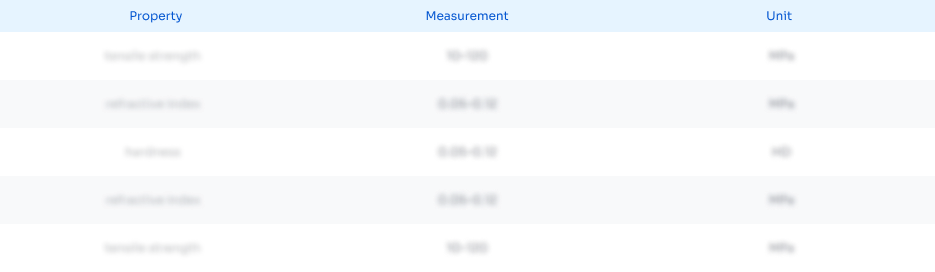
Abstract
Description
Claims
Application Information
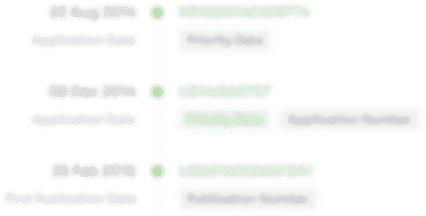
- R&D Engineer
- R&D Manager
- IP Professional
- Industry Leading Data Capabilities
- Powerful AI technology
- Patent DNA Extraction
Browse by: Latest US Patents, China's latest patents, Technical Efficacy Thesaurus, Application Domain, Technology Topic, Popular Technical Reports.
© 2024 PatSnap. All rights reserved.Legal|Privacy policy|Modern Slavery Act Transparency Statement|Sitemap|About US| Contact US: help@patsnap.com