Black box antagonistic attack defense method based on sample selection and model evolution
An adversarial and sample-based technology, applied in character and pattern recognition, instruments, computer components, etc., can solve problems such as different attack effects
- Summary
- Abstract
- Description
- Claims
- Application Information
AI Technical Summary
Problems solved by technology
Method used
Image
Examples
Embodiment Construction
[0058] The present invention will be further described below in conjunction with the accompanying drawings.
[0059] refer to Figure 1 ~ Figure 3 , a black-box adversarial attack defense method based on sample selection and model evolution, including the following steps:
[0060] 1) Generate an adversarial sample, the process is as follows:
[0061] 1.1) Initialization parameter i=1; if training for the first time, set the number of samples in the adversarial sample pool m0=0, and the number of samples selected in the sample selector Ss Num 0 =Num 1 =···=Num n = a, a is a constant;
[0062] 1.2) Use the sample selector Ss to randomly select samples corresponding to the number Num from the sample S; if the number of j-type samples m j Greater than the number Num to be selected for this class j , then only choose m j samples;
[0063] 1.3) Input the obtained samples into the i-th attack model A i , get the corresponding adversarial sample A i S;
[0064] 1.4) i=i+1, ...
PUM
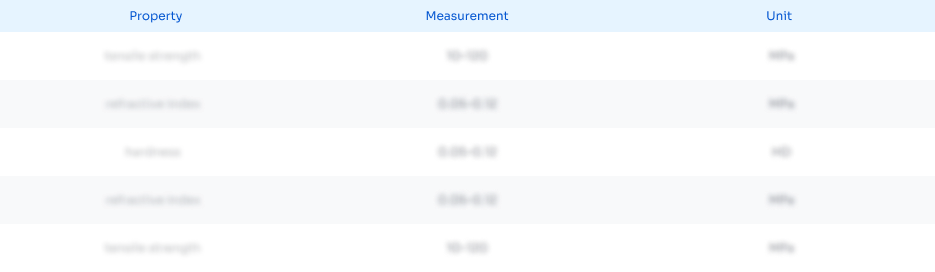
Abstract
Description
Claims
Application Information
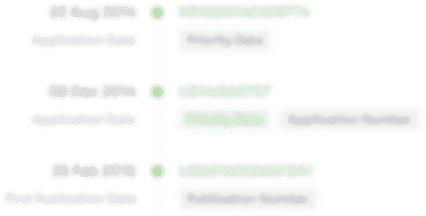
- R&D Engineer
- R&D Manager
- IP Professional
- Industry Leading Data Capabilities
- Powerful AI technology
- Patent DNA Extraction
Browse by: Latest US Patents, China's latest patents, Technical Efficacy Thesaurus, Application Domain, Technology Topic, Popular Technical Reports.
© 2024 PatSnap. All rights reserved.Legal|Privacy policy|Modern Slavery Act Transparency Statement|Sitemap|About US| Contact US: help@patsnap.com