Full connection neural network-based classification method of low interception radar signals
A neural network and radar signal technology, applied in biological neural network model, neural architecture, reflection/re-radiation of radio waves, etc., can solve only 64.8%, can not achieve classification well, can not meet low interception radar signal resolution rate requirements and other issues to achieve the effect of improving the accuracy rate
- Summary
- Abstract
- Description
- Claims
- Application Information
AI Technical Summary
Problems solved by technology
Method used
Image
Examples
Embodiment Construction
[0026] The implementation of the present invention will be further described in detail below in conjunction with the accompanying drawings.
[0027] refer to figure 1 , the implementation steps of the present invention are as follows:
[0028] Step 1: Generate a low intercept radar signal.
[0029] This implementation generates 300,000 low-interception radar signals through simulation, and divides these signals into the following 6 categories:
[0030] The first type of signal is the bpsk signal, the second type of signal is the costas signal, and the third type of signal is the frank signal.
[0031] The fourth type of signal is the LFM code signal, the fifth type of signal is the fmcw signal, and the sixth type of signal is the SLFM signal, wherein the first type of bpsk signal, the second type of costas signal and the third type of frank code signal are phase modulation signals, The fourth type of LFM signal, the fifth type of fmcw signal and the sixth type of SLFM signa...
PUM
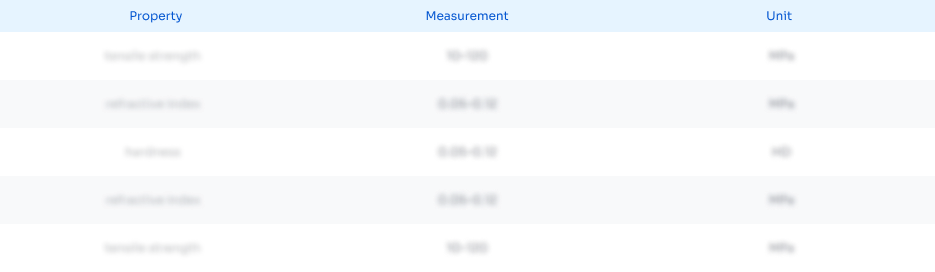
Abstract
Description
Claims
Application Information
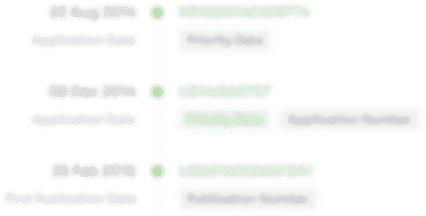
- R&D
- Intellectual Property
- Life Sciences
- Materials
- Tech Scout
- Unparalleled Data Quality
- Higher Quality Content
- 60% Fewer Hallucinations
Browse by: Latest US Patents, China's latest patents, Technical Efficacy Thesaurus, Application Domain, Technology Topic, Popular Technical Reports.
© 2025 PatSnap. All rights reserved.Legal|Privacy policy|Modern Slavery Act Transparency Statement|Sitemap|About US| Contact US: help@patsnap.com