Convolutional neural network structure-based traffic flow prediction method
A convolutional neural network and network structure technology, applied in the field of traffic prediction, can solve the problem that the shallow model cannot mine traffic flow data information well, and achieve the effect of improving prediction accuracy and high prediction accuracy.
- Summary
- Abstract
- Description
- Claims
- Application Information
AI Technical Summary
Problems solved by technology
Method used
Image
Examples
example
[0094] Example: A traffic flow prediction method based on convolutional neural network structure, including the following steps:
[0095] 1) Select experimental data
[0096] The original traffic flow data set contains 14 days' traffic flow data (i.e. p=10, q=720x14) of 10 road sections. The interval T is 2min.
[0097] The road traffic flow data of 10 road sections in the first 10 days are used as the training data set for model parameter training. The road traffic flow data of 4 days after 10 road sections is used as the experimental data set to verify the algorithm.
[0098] 2) Parameter determination
[0099] In the process of building a convolutional neural network model, the main parameters involved are: the number of road sections N, the number of historical traffic flow data n, the number of single-batch training samples m, the number of channels k, and the convolutional layer weight matrix W 1 , Convolution layer bias item b 1 , Reshape layer parameter size, full...
PUM
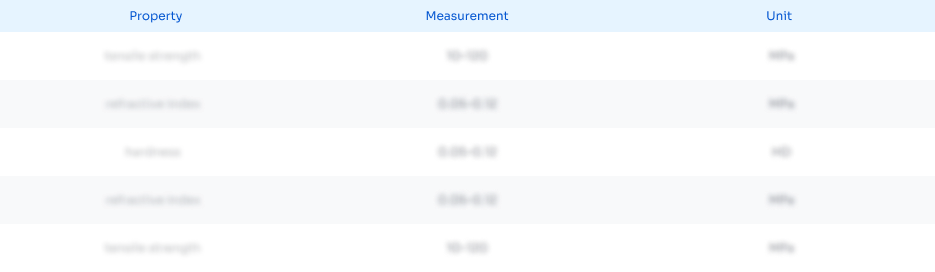
Abstract
Description
Claims
Application Information
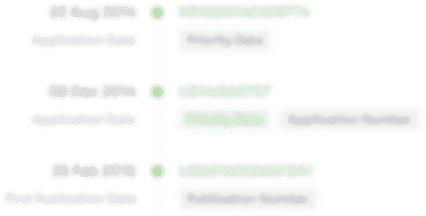
- R&D
- Intellectual Property
- Life Sciences
- Materials
- Tech Scout
- Unparalleled Data Quality
- Higher Quality Content
- 60% Fewer Hallucinations
Browse by: Latest US Patents, China's latest patents, Technical Efficacy Thesaurus, Application Domain, Technology Topic, Popular Technical Reports.
© 2025 PatSnap. All rights reserved.Legal|Privacy policy|Modern Slavery Act Transparency Statement|Sitemap|About US| Contact US: help@patsnap.com