Parameter quantization method of spiking neural network (SNN)
A technology of spiking neural network and neural network, which is applied in neural learning methods, biological neural network models, physical implementation, etc. On-chip resource consumption and computational complexity, the effect of improving computational speed and saving storage resources
- Summary
- Abstract
- Description
- Claims
- Application Information
AI Technical Summary
Problems solved by technology
Method used
Image
Examples
Embodiment Construction
[0027] The present invention will be described in detail below in conjunction with the accompanying drawings, so that those skilled in the art can better understand the present invention. It should be noted that in the following description, when detailed descriptions of known functions and designs may dilute the main content of the present invention, these descriptions will be omitted here.
[0028] Such as figure 1 Shown, a kind of SNN parameter quantization method, comprises the following steps:
[0029] S1: Obtain the original SNN that has been trained.
[0030] The neuron in the SNN is a pulse neuron (such as a LIF neuron), which has the function of integrating and accumulating input pulses and the function of emitting pulses. The SNN uses pulse sequences as input. The main parameters of the SNN include weights, thresholds, leakage constants, set voltages, Refractory period, synaptic delay, etc. The trained SNN has high-precision classification, recognition and other f...
PUM
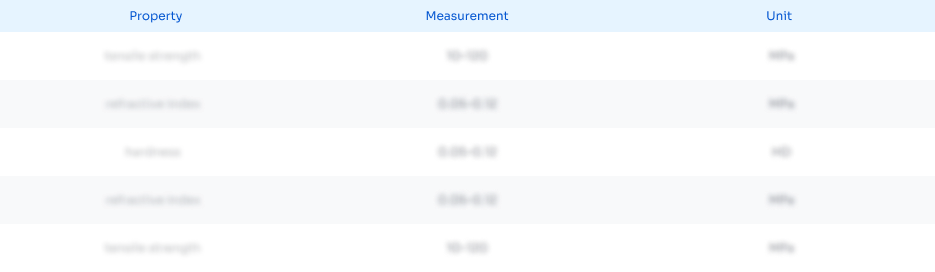
Abstract
Description
Claims
Application Information
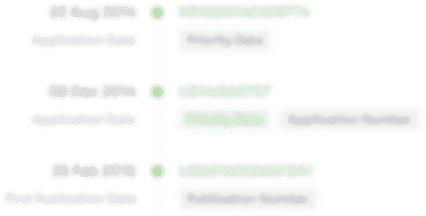
- R&D
- Intellectual Property
- Life Sciences
- Materials
- Tech Scout
- Unparalleled Data Quality
- Higher Quality Content
- 60% Fewer Hallucinations
Browse by: Latest US Patents, China's latest patents, Technical Efficacy Thesaurus, Application Domain, Technology Topic, Popular Technical Reports.
© 2025 PatSnap. All rights reserved.Legal|Privacy policy|Modern Slavery Act Transparency Statement|Sitemap|About US| Contact US: help@patsnap.com