High-resolution image prediction method based on loss function constructed considering image texture information
A technology of high-resolution images and low-resolution images, applied in image prediction and image prediction based on deep learning, can solve the problems of too smooth important details, inability to measure changes and differences in image information, insufficient detail features of super-resolution images and problems Obvious problems, to achieve the effect of practical application value and performance improvement
- Summary
- Abstract
- Description
- Claims
- Application Information
AI Technical Summary
Problems solved by technology
Method used
Image
Examples
Embodiment Construction
[0035] Below in conjunction with accompanying drawing, technical scheme of the present invention is described in further detail:
[0036] Those skilled in the art can understand that, unless otherwise defined, all terms (including technical terms and scientific terms) used herein have the same meaning as commonly understood by one of ordinary skill in the art to which this invention belongs. It should also be understood that terms such as those defined in commonly used dictionaries should be understood to have a meaning consistent with the meaning in the context of the prior art, and will not be interpreted in an idealized or overly formal sense unless defined as herein Explanation.
[0037] The present invention constructs a new loss function based on MSE and image variance minimization under the framework of the SRCNN super-resolution algorithm. The loss function proposed by the present invention is based on MSE, and calculates the area texture feature loss according to a c...
PUM
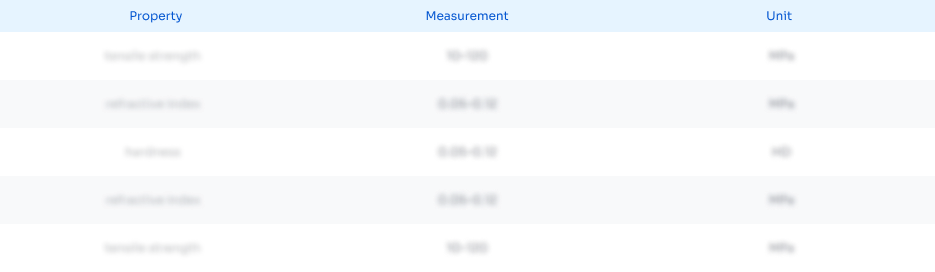
Abstract
Description
Claims
Application Information
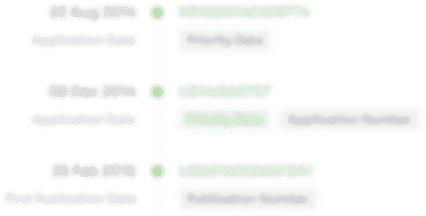
- R&D
- Intellectual Property
- Life Sciences
- Materials
- Tech Scout
- Unparalleled Data Quality
- Higher Quality Content
- 60% Fewer Hallucinations
Browse by: Latest US Patents, China's latest patents, Technical Efficacy Thesaurus, Application Domain, Technology Topic, Popular Technical Reports.
© 2025 PatSnap. All rights reserved.Legal|Privacy policy|Modern Slavery Act Transparency Statement|Sitemap|About US| Contact US: help@patsnap.com