Motor group fault diagnosis method based on binary deep neural network
A deep neural network and fault diagnosis technology, applied in the field of training fault diagnosis model using binary deep neural network, fault diagnosis of large-scale motor groups, and real-time fault diagnosis of large-scale motor groups, which can solve the fault diagnosis of large-scale motor groups and other problems to achieve the effect of saving forward propagation time, reducing costs, and saving data storage space
- Summary
- Abstract
- Description
- Claims
- Application Information
AI Technical Summary
Problems solved by technology
Method used
Image
Examples
Embodiment
[0054] The present invention uses the proposed method and the mainstream deep neural network and traditional machine learning methods to carry out fault diagnosis experiment verification on a certain type of motor. Table 1 shows part of the attribute data. The data consists of four types of faults, each of which includes 850 training samples and 120 testing samples.
[0055] Table 1 Partial fault attribute data
[0056]
[0057] First, column standardize the data, reduce all data to [-1,1], and then perform One-Hot encoding on the output data according to the fault type. For example, currently there are five types of fault data, the One-Hot code of the first type of fault is [1,0,0,0], and the One-Hot code of the third type of fault is [0,0,1,0].
[0058] Secondly, the principal component analysis method is used to reduce the dimensionality of the original 97-dimensional fault data, and the eigenvalues with a cumulative contribution rate of 85% are taken to reduce the da...
PUM
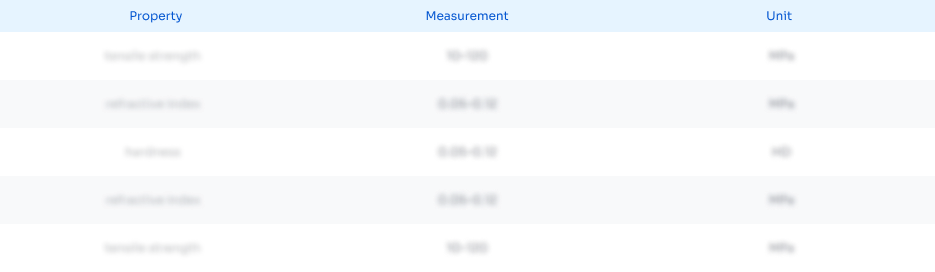
Abstract
Description
Claims
Application Information
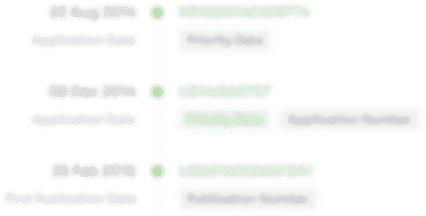
- R&D Engineer
- R&D Manager
- IP Professional
- Industry Leading Data Capabilities
- Powerful AI technology
- Patent DNA Extraction
Browse by: Latest US Patents, China's latest patents, Technical Efficacy Thesaurus, Application Domain, Technology Topic, Popular Technical Reports.
© 2024 PatSnap. All rights reserved.Legal|Privacy policy|Modern Slavery Act Transparency Statement|Sitemap|About US| Contact US: help@patsnap.com