CNN multipoint regression prediction model for traffic flow prediction
A technology of convolutional neural network and traffic flow, which is applied in the field of multi-point regression prediction model, achieves the effect of time complexity and feature selection, and avoids the disappearance of spatial information
- Summary
- Abstract
- Description
- Claims
- Application Information
AI Technical Summary
Problems solved by technology
Method used
Image
Examples
Embodiment 1
[0056] Such as figure 1 with figure 2 As shown, a convolutional neural network multi-point regression forecasting model for traffic flow forecasting includes the following steps:
[0057] The first perceptual input layer: the input of training data, which usually needs to be converted into matrix form;
[0058] The second convolutional layer: Convolve the input layer data and output it after passing the activation function;
[0059] The third convolutional layer: Convolve the output of the previous layer as input, and output it after passing the activation function; the number of convolutional layers is determined according to the actual effect, and more convolutional layers cannot guarantee the performance of the network model. Improvement. The third layer is the best result after our experiment. For this convolutional layer, it can also include the fourth convolutional layer, the fifth convolutional layer, and other convolutional layers.
[0060] The fourth full link lay...
Embodiment 2
[0091] The implementation steps of traffic state prediction for expressways in Shanghai are as follows:
[0092] 1) Based on the data of the Shanghai expressway ground induction coil, the space-time relationship of the coil is divided into different section types: ordinary section, ramp section, diversion section, weaving section section, and confluence section;
[0093] 2) Calibrate the congestion nodes through the TSI index of Shanghai:
[0094]
[0095] Among them, h represents the actual vehicle speed, f represents the free flow speed; TSI identifies the congested node;
[0096] Table 1 Road traffic status corresponding to different index intervals
[0097]
[0098] 3) Carry out the sensitivity analysis of the feature, take the feature samples with different numbers of nodes upstream and different numbers of nodes downstream of the target point to predict the final target point; enable the multi-point regression prediction model of the six-layer non-pooling convolut...
PUM
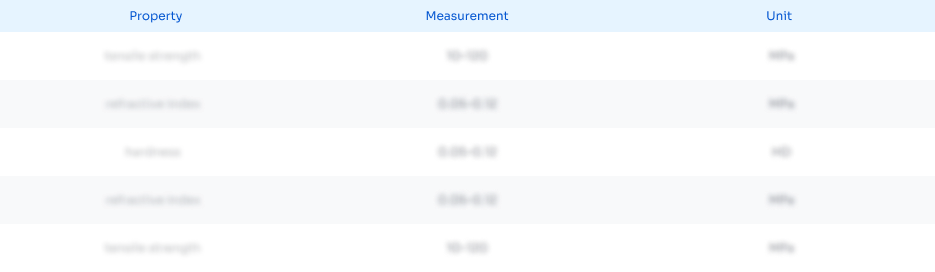
Abstract
Description
Claims
Application Information
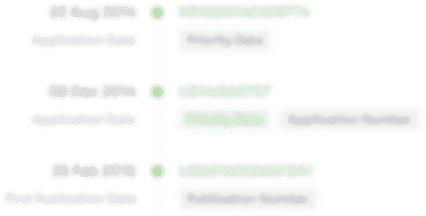
- R&D
- Intellectual Property
- Life Sciences
- Materials
- Tech Scout
- Unparalleled Data Quality
- Higher Quality Content
- 60% Fewer Hallucinations
Browse by: Latest US Patents, China's latest patents, Technical Efficacy Thesaurus, Application Domain, Technology Topic, Popular Technical Reports.
© 2025 PatSnap. All rights reserved.Legal|Privacy policy|Modern Slavery Act Transparency Statement|Sitemap|About US| Contact US: help@patsnap.com