Unsupervised remote sensing image semantic segmentation method based on super-resolution and domain self-adaption
A super-resolution, remote sensing image technology, applied in image analysis, image enhancement, image data processing, etc., can solve the problems of pixel-level positioning information loss, unbalanced types, etc., to avoid explosion and disappearance, strong robustness, The effect of alleviating the category imbalance problem
- Summary
- Abstract
- Description
- Claims
- Application Information
AI Technical Summary
Problems solved by technology
Method used
Image
Examples
Embodiment Construction
[0091] like Figure 1 to Figure 9 As shown, the present invention based on super-resolution and domain adaptive unsupervised remote sensing image semantic segmentation method replaces the ASPP module in the original super-resolution domain adaptive unsupervised remote sensing image semantic segmentation method through the feature pyramid attention module, and the residual The difference feature pyramid attention module is applied to the discriminator to obtain accurate pixel-level attention for high-level semantic features; the problem of category imbalance is alleviated through the Dice coefficient loss function, which includes the following steps:
[0092] Step 1: Obtain a low-resolution remote sensing image dataset in the source domain and a high-resolution remote sensing image dataset in the target domain. The source domain and target domain remote sensing image datasets are obtained through remote sensing satellites; the source domain data includes low-resolution original ...
PUM
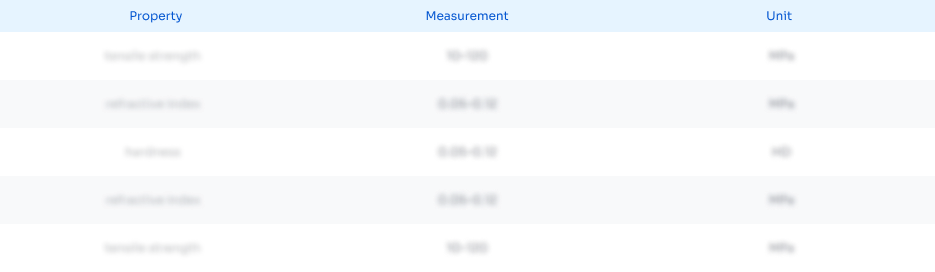
Abstract
Description
Claims
Application Information
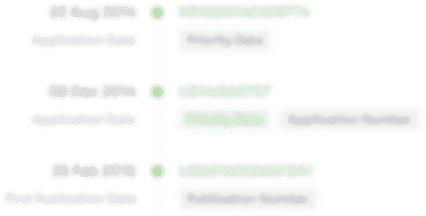
- R&D Engineer
- R&D Manager
- IP Professional
- Industry Leading Data Capabilities
- Powerful AI technology
- Patent DNA Extraction
Browse by: Latest US Patents, China's latest patents, Technical Efficacy Thesaurus, Application Domain, Technology Topic.
© 2024 PatSnap. All rights reserved.Legal|Privacy policy|Modern Slavery Act Transparency Statement|Sitemap