Sparsity Adaptive Variable Step Size Matching and Pursuit Method Based on Compressed Sensing
A compressed sensing and matching tracking technology, applied in code conversion, electrical components, etc., can solve the problems of long reconstruction time, overestimation or underestimation, and unimproved reconstruction time of the SAMP method, achieving reconstruction accuracy and computational complexity. High degree of consideration, avoid overestimation and underestimation, reduce the effect of computational complexity
- Summary
- Abstract
- Description
- Claims
- Application Information
AI Technical Summary
Problems solved by technology
Method used
Image
Examples
Embodiment 1
[0150] Example 1. Estimate the initial value K of the sparsity degree with the estimation module of the initial value of the degree of sparsity 0 .
[0151] A) Input measurement matrix Φ, observed signal y, RIP parameter δ P , using a Gaussian sparse signal with a mean value of 1 and a variance of 0, the length of the signal is N=256, the degree of sparsity P=44, and the number of measurements M=128.
[0152] B) Estimate the initial value of sparsity K' with SAVSMP method 0 . δ P The initial value K' of the sparsity estimated when taking 0.1, 0.15, 0.25, 0.35 respectively 0 Such as Figure 4 shown. Visible δ P When taking a smaller value, the estimated K'0 Larger, often in an overestimated state, when δ P When taking a larger value, the estimated K' 0 Small and often underestimated.
[0153] C) Use the SAVSMP method to adjust the initial value of the sparsity K' 0 Perform adaptive processing to get K 0 , using the AStMP method to process the sparsity estimate K 0 ...
Embodiment 2
[0154] Embodiment 2. Using the step size setting module to adaptively set the step size.
[0155] A) Input measurement matrix Φ, observed signal y, RIP parameter δ P , using a Gaussian sparse signal with a mean value of 1 and a variance of 0, the length of the signal is N=256, the degree of sparsity P=44, the number of measurements M=128, and the parameter σ 1 =1×10 -6 ,σ 2 =10, weak matching parameter τ=0.9.
[0156] B) Use the SAVSMP method to set the large step size obtained by the exponential estimation method to approximate the real sparsity, and the step size change coefficient is recorded as β, and the change curve is as follows Image 6 shown.
[0157] C) Use the SAVSMP method to set the small step size obtained by the weak matching method to approximate the real sparsity.
[0158] D) Update the step size K with the SAVSMP method j .
Embodiment 3
[0159] Example 3. Comparison of SAMP method, SASP method, AStMP method, and SAVSMP method for estimating the sparsity K.
[0160] A) Input measurement matrix Φ, observed signal y, RIP parameter δ P , using a Gaussian sparse signal with a mean value of 1 and a variance of 0, the length of the signal is N=256, the degree of sparsity P=44, the number of measurements M=128, and the parameter σ 1 =1×10 -6 ,σ 2 =10, weak matching parameter τ=0.9.
[0161] B) Set the step size L=4, use the SAMP method to estimate the sparsity K, the relationship curve between the number of iterations and the estimated sparsity K is as follows Figure 7 shown. It can be seen that the SAMP method has a large number of iterations, and it takes about 22 iterations to estimate the sparsity.
[0162] C) Setting δ P = 0.1, using the SASP method to estimate the sparsity K, the relationship curve between the number of iterations and the estimated sparsity K is as follows Figure 7 shown. It can be see...
PUM
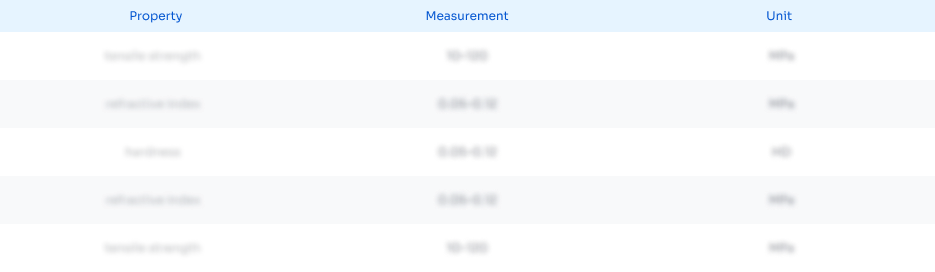
Abstract
Description
Claims
Application Information
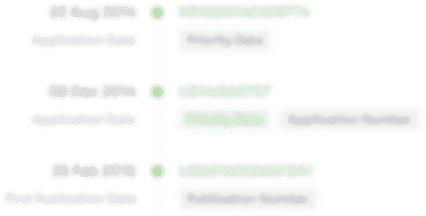
- R&D
- Intellectual Property
- Life Sciences
- Materials
- Tech Scout
- Unparalleled Data Quality
- Higher Quality Content
- 60% Fewer Hallucinations
Browse by: Latest US Patents, China's latest patents, Technical Efficacy Thesaurus, Application Domain, Technology Topic, Popular Technical Reports.
© 2025 PatSnap. All rights reserved.Legal|Privacy policy|Modern Slavery Act Transparency Statement|Sitemap|About US| Contact US: help@patsnap.com