Spectrum sensing method based on alpha-divergence of information geometry
An information geometry and spectrum sensing technology, applied in the field of wireless communication, can solve the problems of being easily affected by noise fluctuations, reducing system sensitivity, and high complexity, achieving a simple and practical calculation process, good spectrum sensing stability, and good spectrum sensing efficiency. Effect
- Summary
- Abstract
- Description
- Claims
- Application Information
AI Technical Summary
Problems solved by technology
Method used
Image
Examples
no. 1 example
[0047] This embodiment is the first embodiment of the spectrum sensing method based on α-divergence of information geometry, including the following steps:
[0048] S10. Establish a cognitive radio model, and generate N noise covariance matrices R for noise environment simulation k , and take one of them as the unit R to be detected;
[0049] S20. Using the natural gradient descent algorithm to calculate the N noise covariance matrices R in step S10 k Riemann mean
[0050] S30. Calculate the detection unit R in step S10 and the Riemann mean value in step S20 The geometric distance L between
[0051] S40. Set false alarm probability P f , repeat steps S10 to S30 n times in total to obtain n geometric distances L, sort the obtained n geometric distances L in descending order, and calculate the threshold T;
[0052] S50. Perform data sampling on the primary user signal to obtain a known signal matrix, generate several unknown signal covariance matrices through simulation,...
Embodiment 2
[0080] This embodiment is an application embodiment of the spectrum sensing method based on the α-divergence of information geometry in Embodiment 1: In this embodiment, the false alarm probability of a given condition is P fa =0.01, the sampling frequency is P fs =1000, the number of sampling points ns=4000, the α divergence of the information geometry of the present embodiment and the maximum and minimum eigenvalue energy spectrum sensing (MME) are simulated respectively, and the simulation results are as follows figure 2 shown. Depend on figure 2 It can be seen that as the SNR increases, the perceptual performance of the two algorithms improves rapidly, and the perception effect of the α-divergence of the information geometry improves more significantly; when the SNR is the same, the α-divergence based on the information set The detection efficiency is also higher than that of the MME algorithm.
PUM
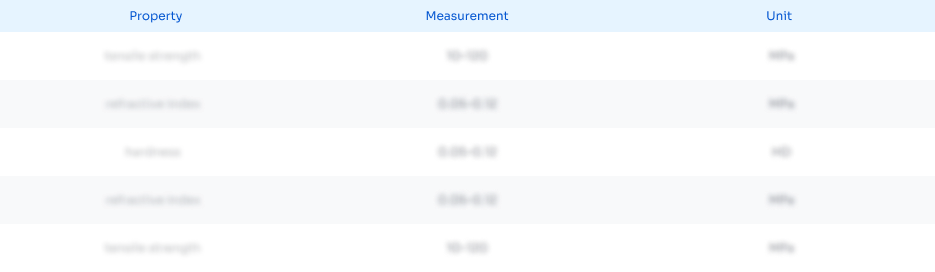
Abstract
Description
Claims
Application Information
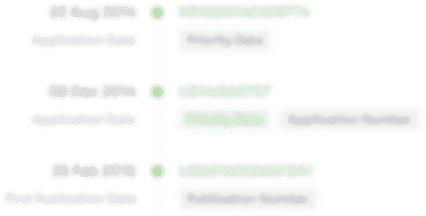
- R&D Engineer
- R&D Manager
- IP Professional
- Industry Leading Data Capabilities
- Powerful AI technology
- Patent DNA Extraction
Browse by: Latest US Patents, China's latest patents, Technical Efficacy Thesaurus, Application Domain, Technology Topic, Popular Technical Reports.
© 2024 PatSnap. All rights reserved.Legal|Privacy policy|Modern Slavery Act Transparency Statement|Sitemap|About US| Contact US: help@patsnap.com