Deep clustering method facing single particle cryo-electron microscope images
A technology of cryo-electron microscopy and clustering methods, applied in the field of machine learning, can solve the problems of high time consumption and low accuracy of classification technology, and achieve the effect of improving classification accuracy and noise reduction ability
- Summary
- Abstract
- Description
- Claims
- Application Information
AI Technical Summary
Problems solved by technology
Method used
Image
Examples
Embodiment Construction
[0038] In order to make the technical solutions in this application better understood, the following will describe the application clearly and in detail with reference to the drawings and specific implementations in the embodiments of the application:
[0039] A. Autoencoder based on convolutional neural network to extract particle image features
[0040] An autoencoder based on a convolutional neural network is a method that uses a convolutional neural network to reduce the dimensionality of the input image and restore the original image. The features of the image can be output through the hidden layer network of the self-encoder based on the convolutional neural network. The single-particle cryo-electron microscope image has a strong noise signal. In order to avoid the interference of noise on feature extraction, the present invention uses particle images without noise signals to pre-train the network. Add noise signals such as Gaussian noise and white noise to the input noise-f...
PUM
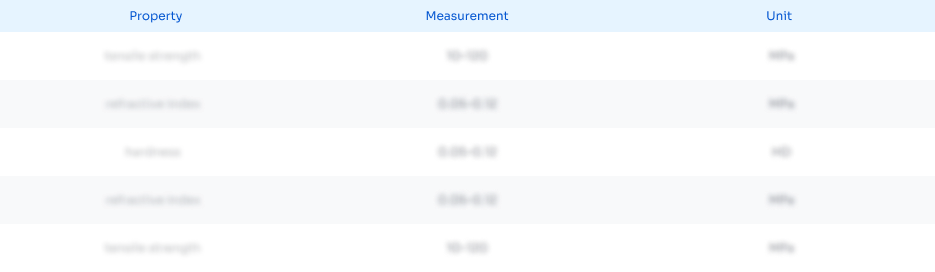
Abstract
Description
Claims
Application Information
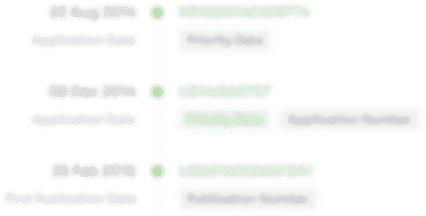
- R&D Engineer
- R&D Manager
- IP Professional
- Industry Leading Data Capabilities
- Powerful AI technology
- Patent DNA Extraction
Browse by: Latest US Patents, China's latest patents, Technical Efficacy Thesaurus, Application Domain, Technology Topic, Popular Technical Reports.
© 2024 PatSnap. All rights reserved.Legal|Privacy policy|Modern Slavery Act Transparency Statement|Sitemap|About US| Contact US: help@patsnap.com