Data stream anomaly detection system based on empirical features and convolution neural network
A convolutional neural network and anomaly detection technology, applied in the field of information security, can solve problems such as large amount of calculation and processing time, consume a lot of computing resources, and the model does not conform to the new data distribution, so as to improve the detection effect and processing efficiency Effect
- Summary
- Abstract
- Description
- Claims
- Application Information
AI Technical Summary
Problems solved by technology
Method used
Image
Examples
Embodiment
[0070] Step 1: Perform data preprocessing on the network data stream, and divide the original mass packets into data streams. For details, see figure 1 .
[0071] According to the five-tuple information (protocol, source address, destination address, source port number, destination port number) of the original message header, the original message with the same five-tuple information and within a certain period of time is aggregated into flow data. Step 2: The empirical feature extraction module extracts artificial empirical features from the data stream, see figure 2 .
[0072] (1) Query the data flow statistical information database to obtain the effective statistics of the data flow layer for detecting abnormal data flow, such as the port number of the four-layer protocol, the number of packets of the flow, the size of the packet, and the time interval between packets, etc. features are extracted.
[0073] (2) Query the packet header information database to obtain the se...
PUM
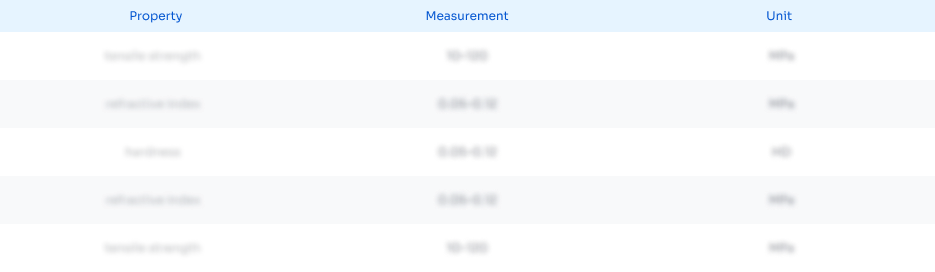
Abstract
Description
Claims
Application Information
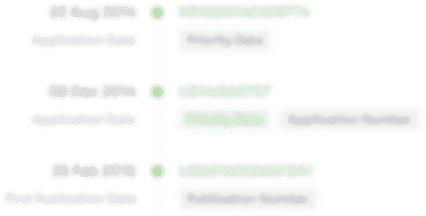
- R&D
- Intellectual Property
- Life Sciences
- Materials
- Tech Scout
- Unparalleled Data Quality
- Higher Quality Content
- 60% Fewer Hallucinations
Browse by: Latest US Patents, China's latest patents, Technical Efficacy Thesaurus, Application Domain, Technology Topic, Popular Technical Reports.
© 2025 PatSnap. All rights reserved.Legal|Privacy policy|Modern Slavery Act Transparency Statement|Sitemap|About US| Contact US: help@patsnap.com