A method for detecting abnormal behavior of electricity consumption of consumers based on isolated forests
A detection method and technology for electrical anomalies, which are applied in data processing applications, electrical digital data processing, digital data information retrieval, etc., can solve the problems of large subsequent operations and long running time of analysis and computing, so as to improve computing speed and reduce computing data. , to avoid the effect of the crash phenomenon
- Summary
- Abstract
- Description
- Claims
- Application Information
AI Technical Summary
Problems solved by technology
Method used
Image
Examples
Embodiment 1
[0033] A method for detecting abnormal power consumption behavior of users based on isolated forest, comprising the following steps:
[0034] S1. Obtain power consumption time series data through data collection;
[0035] S2. Clean the data to remove incomplete data, wrong data, and duplicate data;
[0036] S3, feature extraction based on statistics:
[0037] S31. Data definition: S311. Let the data set be X={x n}, n ranges from 1 to N, the data set contains N daily electricity users, and each user is divided into D days, M months, and Q quarters of electricity consumption data; S312, the daily electricity consumption sequence of each user: x n ={x nd}, d ranges from 1 to D; S313, the monthly power consumption sequence of each user: y n ={y nm}, m takes 1 to M, S114. Quarterly power consumption sequence of each user: z n ={z nq}, q takes 1 to Q,
[0038] S32. Divide the user's electricity consumption behavior characteristics in units of year, quarter, and month in...
Embodiment 2
[0113] The only difference between this embodiment and Embodiment 1 is that this embodiment only changes the preprocessing model on the basis of Embodiment 1, and this embodiment uses an automatic encoder.
[0114] First, build a traditional single hidden layer autoencoder model, which is a fully connected neural network, such as figure 2 shown.
[0115] figure 2 In , the first half of the model is used as the automatic encoding part, and the second half is used as the automatic decoding part. The model takes the 334 feature dimensions cleaned from the original data as input and output at the same time, that is, the number of neurons in the input layer is the same as the number of neurons in the output layer. Here, the number of nodes in the middle layer is set to 32, which is less than the number of nodes in the input layer and output layer, which plays the role of data compression.
[0116] Next, configure the relevant parameters for the autoencoder model. Among them, ...
Embodiment 3
[0124] The difference between this embodiment and Embodiment 2 is that this embodiment only adds a hidden layer to the automatic encoder on the basis of Embodiment 2.
[0125] The previous autoencoder data processing model only established a single hidden layer. This time, a deeper autoencoder model was established for the data to be processed. The network structure is as follows: Figure 5 Shown:
[0126] The basic configuration parameters are the same as the previous model configuration. The training optimization function of the configuration model is adadelta, the loss function is binary_crossentropy, the number of training is 100 times, and the activation function of the middle encoding layer and decoding layer uses the ReLU activation function. The software algorithm such as Figure 7 shown.
PUM
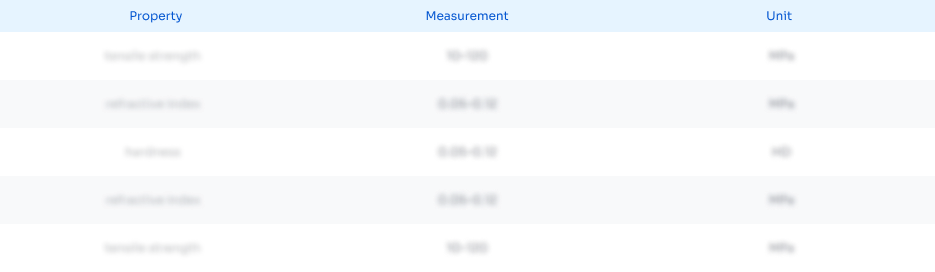
Abstract
Description
Claims
Application Information
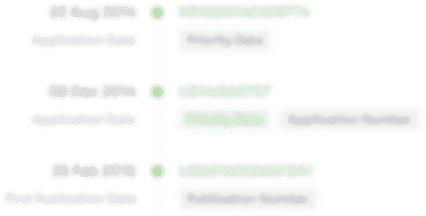
- R&D Engineer
- R&D Manager
- IP Professional
- Industry Leading Data Capabilities
- Powerful AI technology
- Patent DNA Extraction
Browse by: Latest US Patents, China's latest patents, Technical Efficacy Thesaurus, Application Domain, Technology Topic, Popular Technical Reports.
© 2024 PatSnap. All rights reserved.Legal|Privacy policy|Modern Slavery Act Transparency Statement|Sitemap|About US| Contact US: help@patsnap.com