Facial expression recognition method based on joint learning identity information and emotion information
A facial expression and identity information technology, applied in the field of computer vision and affective computing, to achieve the effect of performance improvement, significant system performance, and improved robustness
- Summary
- Abstract
- Description
- Claims
- Application Information
AI Technical Summary
Problems solved by technology
Method used
Image
Examples
Embodiment 1
[0037] Embodiment one: use the present invention to test on Extended Cohn-Kanade (CK+) data.
[0038] Step 1: First use the CASIA-WebFace face recognition database to train the sub-network used to extract face identity information. CASIA-WebFace contains a total of 494,414 images of 10,757 individuals. At the same time, use the LabeledFaces in the Wild (LFW) dataset to evaluate the accuracy of face recognition. The structure of the sub-network includes multiple convolutional layers and pooling layers, and finally a 160-dimensional feature vector of face identity information can be extracted. After training and tuning, the network can achieve 91% accuracy on the LFW dataset. Since our ultimate goal is not to perform face verification, we do not optimize the face verification performance too much.
[0039] Step 2: Use the CK+ facial expression database to train the sub-network used to extract facial expression information. The CK+ database contains 327 image sequences with f...
Embodiment 2
[0042] Embodiment two: use the technology of the present invention to test on FER+ data
[0043] In the first step, consistent with Embodiment 1, first use the CASIA-WebFace face recognition database to train a sub-network for extracting face identity information. CASIA-WebFace contains a total of 494,414 images of 10,757 individuals. At the same time, use the Labeled Faces in the Wild (LFW) dataset to evaluate the accuracy of face recognition. The structure of the sub-network includes multiple convolutional layers and pooling layers, and finally a 160-dimensional feature vector of face identity information can be extracted. After training and tuning, the network can achieve 91% accuracy on the LFW dataset. Since our ultimate goal is not to perform face verification, we do not optimize the face verification performance too much.
[0044] In the second step, since the amount of data in the FER+ dataset has increased significantly compared to the CK+ dataset, the ResNet 18-la...
PUM
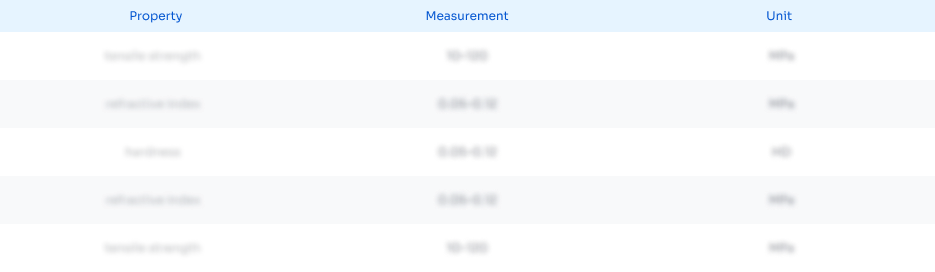
Abstract
Description
Claims
Application Information
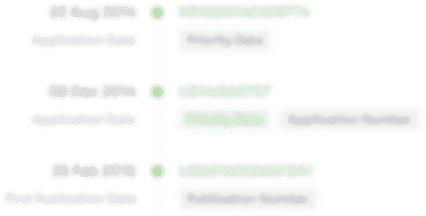
- R&D
- Intellectual Property
- Life Sciences
- Materials
- Tech Scout
- Unparalleled Data Quality
- Higher Quality Content
- 60% Fewer Hallucinations
Browse by: Latest US Patents, China's latest patents, Technical Efficacy Thesaurus, Application Domain, Technology Topic, Popular Technical Reports.
© 2025 PatSnap. All rights reserved.Legal|Privacy policy|Modern Slavery Act Transparency Statement|Sitemap|About US| Contact US: help@patsnap.com