A SAR image target recognition method based on denoising self-coding network
A target recognition and image technology, which is applied in the field of SAR image target recognition based on denoising self-encoding network, can solve the problems that the SAR image target recognition algorithm is not general enough, time-consuming, not stable and robust enough, etc.
- Summary
- Abstract
- Description
- Claims
- Application Information
AI Technical Summary
Problems solved by technology
Method used
Image
Examples
Embodiment approach
[0038] Such as figure 1 Shown, the SAR image target recognition algorithm principle of the present invention of the present invention is:
[0039] (1) First use the three-dimensional block matching algorithm (BM3D) to combine image sub-blocks with similar structures into a three-dimensional array, use the method of joint filtering to filter the image in the transform domain, and finally obtain the denoised image through inverse transformation.
[0040] (2) For the original image and the denoised training image, use the sliding window to extract dense feature points, and store them in pairs according to the corresponding positions. Input for later training of the deep denoising encoder. For the test image, the denoising process is no longer performed, and the dense SIFT features are directly extracted. Assuming the scale S={0, 1, 2}, the sliding window size and the corresponding sampling interval step are:
[0041] win size (s)=16×2 s ,
[0042] win step (s)=8×2 s ,
...
PUM
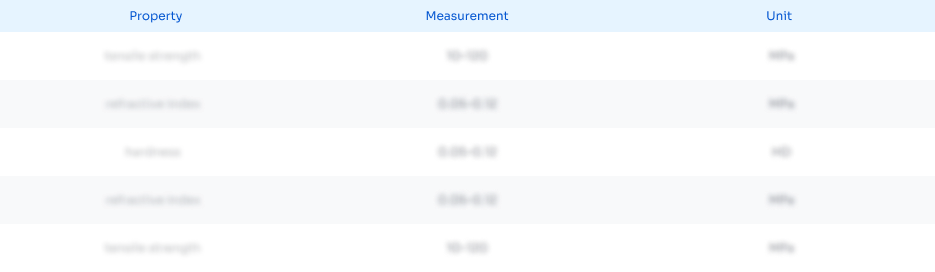
Abstract
Description
Claims
Application Information
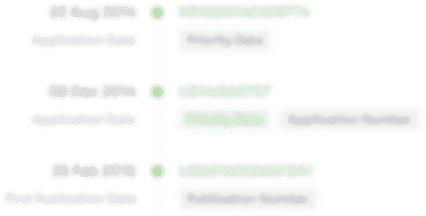
- R&D Engineer
- R&D Manager
- IP Professional
- Industry Leading Data Capabilities
- Powerful AI technology
- Patent DNA Extraction
Browse by: Latest US Patents, China's latest patents, Technical Efficacy Thesaurus, Application Domain, Technology Topic, Popular Technical Reports.
© 2024 PatSnap. All rights reserved.Legal|Privacy policy|Modern Slavery Act Transparency Statement|Sitemap|About US| Contact US: help@patsnap.com