Industrial data nonlinear cause and effect analysis method based on sparse deep neural network
A deep neural network and industrial data technology, applied in the field of fault diagnosis in industrial control systems, can solve the problems of only detecting linear causality and low calculation efficiency, so as to improve algorithm efficiency, reduce the number of inspections, and reduce the number of tests Effect
- Summary
- Abstract
- Description
- Claims
- Application Information
AI Technical Summary
Problems solved by technology
Method used
Image
Examples
Embodiment Construction
[0061] Taking the causal analysis of TE process simulation data as an example, the method of nonlinear causal analysis using sparse neural network is described in detail for the process data with continuous plant-level oscillation in each loop. It should be pointed out that the following examples are intended to facilitate the understanding of the present invention, but do not limit it in any way.
[0062] In the TE process, there are 41 measured variables including measurement noise and 12 manipulated variables (11 valve flow signals and reactor stirring rate signals). The main control objectives include: product flow rate, G component content in the product stream, reactor pressure, reactor liquid level, separator liquid level, stripper liquid level, etc. are kept at the set value. The control strategies of its 19 control loops are as follows: figure 1 And shown in Table 1.
[0063] Table 1
[0064]
[0065]
[0066] Among them, the 18th and 19th control loops are o...
PUM
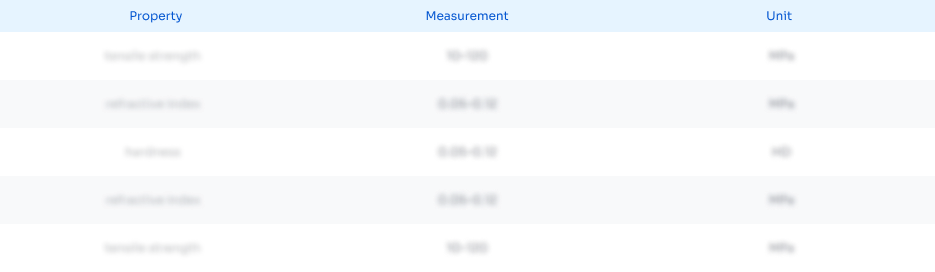
Abstract
Description
Claims
Application Information
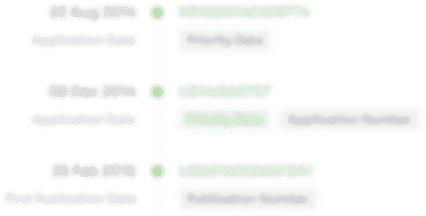
- R&D
- Intellectual Property
- Life Sciences
- Materials
- Tech Scout
- Unparalleled Data Quality
- Higher Quality Content
- 60% Fewer Hallucinations
Browse by: Latest US Patents, China's latest patents, Technical Efficacy Thesaurus, Application Domain, Technology Topic, Popular Technical Reports.
© 2025 PatSnap. All rights reserved.Legal|Privacy policy|Modern Slavery Act Transparency Statement|Sitemap|About US| Contact US: help@patsnap.com