A convolutional neural network model generation method and an image quality optimization method
A convolutional neural network and model generation technology, applied in the field of image processing, can solve problems such as loss of details, amplification of noise, and inflexibility, and achieve the effects of reducing sensitivity, improving robustness, and improving contrast
- Summary
- Abstract
- Description
- Claims
- Application Information
AI Technical Summary
Problems solved by technology
Method used
Image
Examples
Embodiment Construction
[0043] Exemplary embodiments of the present disclosure will be described in more detail below with reference to the accompanying drawings. Although exemplary embodiments of the present disclosure are shown in the drawings, it should be understood that the present disclosure may be embodied in various forms and should not be limited by the embodiments set forth herein. Rather, these embodiments are provided for more thorough understanding of the present disclosure and to fully convey the scope of the present disclosure to those skilled in the art.
[0044] The convolutional neural network model generation method of the present invention is suitable for execution in one or a group of computing devices, that is, the training of the convolutional neural network model is completed in one or a group of computing devices. Computing devices can be, for example, servers (such as web servers, application servers, etc.), personal computers such as desktop computers and notebook computers...
PUM
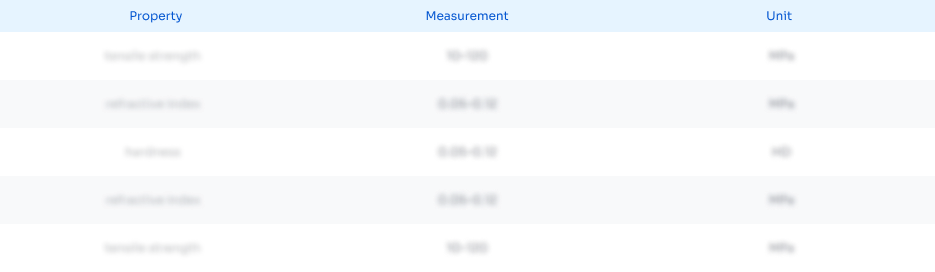
Abstract
Description
Claims
Application Information
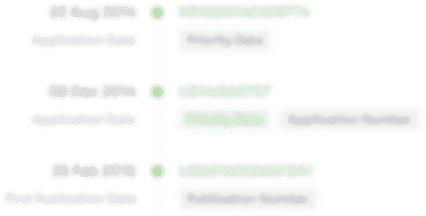
- R&D Engineer
- R&D Manager
- IP Professional
- Industry Leading Data Capabilities
- Powerful AI technology
- Patent DNA Extraction
Browse by: Latest US Patents, China's latest patents, Technical Efficacy Thesaurus, Application Domain, Technology Topic, Popular Technical Reports.
© 2024 PatSnap. All rights reserved.Legal|Privacy policy|Modern Slavery Act Transparency Statement|Sitemap|About US| Contact US: help@patsnap.com