Nuclear localization signal prediction algorithm based on dual-recommendation system of frequent mode and machine learning
A nuclear localization signal, frequent pattern technology, applied in the field of protein biology, can solve the problems of lack of prominence, difficult to mediate nuclear localization signal accuracy and recall, and high redundancy, to achieve improved probability, statistical significance and evolution. effect of meaning
- Summary
- Abstract
- Description
- Claims
- Application Information
AI Technical Summary
Problems solved by technology
Method used
Image
Examples
Embodiment Construction
[0061] In order to make the technical means, creative features, goals and effects achieved by the present invention easy to understand, the present invention will be further described below in conjunction with specific embodiments.
[0062] see figure 1 , figure 2 and image 3 , the nuclear localization signal prediction algorithm based on frequent pattern and machine learning dual recommendation system of the present invention, comprises the following steps:
[0063] S1. Set up a nuclear localization signal training set and a non-nuclear localization signal training set as positive and negative samples respectively. The specific operation is as follows: select 145 NLSs with parental proteins, specific forms, and experimental verification from the NLSdb 2003 and 2017 databases as positive samples, and the negative samples correspond to the length of the positive samples one-to-one and come from the same sequence.
[0064] S2. Using a known word vector model to extract the ...
PUM
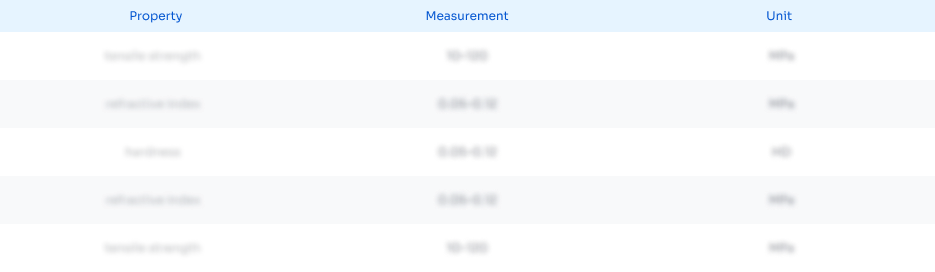
Abstract
Description
Claims
Application Information
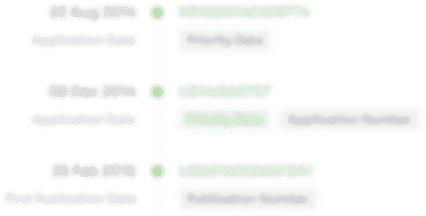
- R&D Engineer
- R&D Manager
- IP Professional
- Industry Leading Data Capabilities
- Powerful AI technology
- Patent DNA Extraction
Browse by: Latest US Patents, China's latest patents, Technical Efficacy Thesaurus, Application Domain, Technology Topic, Popular Technical Reports.
© 2024 PatSnap. All rights reserved.Legal|Privacy policy|Modern Slavery Act Transparency Statement|Sitemap|About US| Contact US: help@patsnap.com