A mobile application malware detection method and system for a power enterprise
A malicious software and mobile application technology, applied in data processing applications, computer components, electrical digital data processing, etc., can solve the problems that support vector machines are not suitable for classification learning, and achieve the effect of reducing learning time and improving learning efficiency
- Summary
- Abstract
- Description
- Claims
- Application Information
AI Technical Summary
Problems solved by technology
Method used
Image
Examples
Embodiment 1
[0071] The invention provides a mobile application malicious software detection method for electric power enterprises. The detection method firstly decompiles the APP sample to obtain the source code of the application, and then uses the static scanning method to extract the feature vectors of the normal application and the malicious application, and constructs the normal and malicious feature sample library. Finally, the machine learning algorithm support vector machine is used to train and learn the feature library, and the SVM classification model for identifying malicious applications is obtained. If there are new samples to be trained, the incremental learning method can be used to quickly learn the features of the new samples without re-learning all the sample features, and finally obtain the iteratively updated SVM classification model.
[0072] The flow chart of mobile application malware detection in electric power enterprises is attached figure 1 As shown, it mainly...
Embodiment 2
[0113] A mobile application malware detection system for electric power enterprises, including:
[0114] Obtaining module: used to obtain the software to be tested, and decompile the software to be tested to obtain the source code of the software to be tested;
[0115] Determining module: used to extract the feature vector of the source code, and input the feature vector of the source code to a pre-built SVM classification model for comparison, and determine whether the software to be detected is malicious software;
[0116] The SVM classification model includes: an SVM classifier, and the SVM classifier is iteratively updated based on a double weight increment method.
[0117] The determination module includes: a model building submodule, a model updating submodule and a determination submodule:
[0118] The model building module is used for: performing feature extraction and constructing an SVM classifier based on a large amount of normal application software and malicious ...
PUM
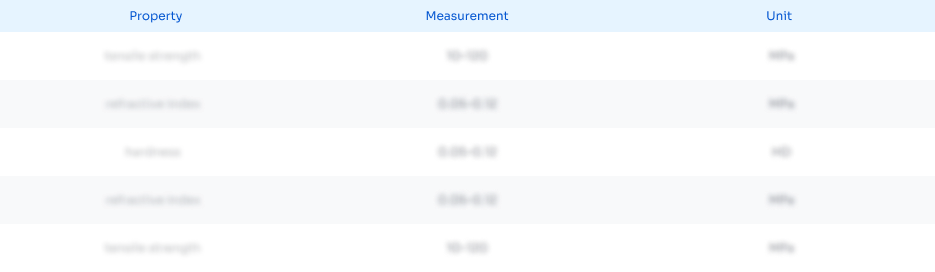
Abstract
Description
Claims
Application Information
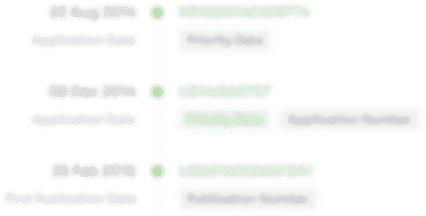
- R&D Engineer
- R&D Manager
- IP Professional
- Industry Leading Data Capabilities
- Powerful AI technology
- Patent DNA Extraction
Browse by: Latest US Patents, China's latest patents, Technical Efficacy Thesaurus, Application Domain, Technology Topic.
© 2024 PatSnap. All rights reserved.Legal|Privacy policy|Modern Slavery Act Transparency Statement|Sitemap