A resource allocation optimization method and system based on reinforcement learning
A technology of reinforcement learning and resource allocation, applied in neural learning methods, biological neural network models, electrical components, etc., can solve problems such as affecting scheduling performance
- Summary
- Abstract
- Description
- Claims
- Application Information
AI Technical Summary
Problems solved by technology
Method used
Image
Examples
Embodiment Construction
[0079] In order to make the object, technical solution and advantages of the present invention clearer, the present invention will be further described in detail below in conjunction with the accompanying drawings and embodiments. It should be understood that the specific embodiments described here are only used to explain the present invention, not to limit the present invention. In addition, the technical features involved in the various embodiments of the present invention described below can be combined with each other as long as they do not constitute a conflict with each other.
[0080] The overall idea of the present invention is to propose a resource allocation optimization method based on reinforcement learning, which first constructs a relevant Markov state transition model based on the characteristics of downlink resource scheduling; A resource scheduling model for reinforcement learning.
[0081] Such as Figure 16 As shown, the resource allocation optimization...
PUM
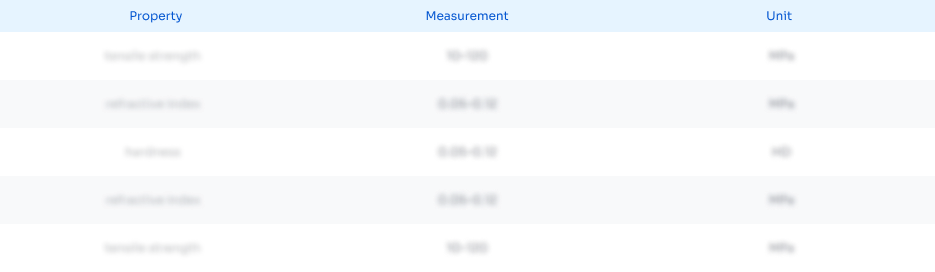
Abstract
Description
Claims
Application Information
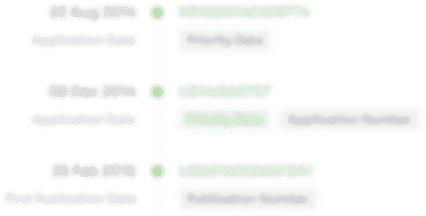
- R&D
- Intellectual Property
- Life Sciences
- Materials
- Tech Scout
- Unparalleled Data Quality
- Higher Quality Content
- 60% Fewer Hallucinations
Browse by: Latest US Patents, China's latest patents, Technical Efficacy Thesaurus, Application Domain, Technology Topic, Popular Technical Reports.
© 2025 PatSnap. All rights reserved.Legal|Privacy policy|Modern Slavery Act Transparency Statement|Sitemap|About US| Contact US: help@patsnap.com