Pipeline defect identification method based on computer vision and machine learning
A technology of computer vision and machine learning, which is applied in computer parts, calculation, character and pattern recognition, etc., can solve the problems of poor anti-interference ability of classification models, influence of environmental changes, low accuracy of pipeline defect recognition, etc., to achieve guaranteed recognition The effect of accuracy, increasing efficiency, and reducing dependence
- Summary
- Abstract
- Description
- Claims
- Application Information
AI Technical Summary
Problems solved by technology
Method used
Image
Examples
Embodiment Construction
[0021] In order to make the object, technical solution and advantages of the present invention clearer, the present invention will be described in further detail below in conjunction with the accompanying drawings and embodiments, which are not intended to limit the present invention.
[0022] Such as figure 1 As shown, the implementation process of the method of the present invention specifically includes the following steps:
[0023] S1010: Carrying a high-definition camera pipeline robot into the pipeline to collect images and video information,
[0024] Gray-scale stretching is performed on the collected pipeline images and videos. Enhance the contrast between the suspected corrosion area and the normal pipeline to make it brighter and darker, and the features are more obvious. The gray value f(x,y) of each pixel (x,y) in the input image is used as the independent variable of the function. The obtained dependent variable is used as the gray value g(x,y) in the output ima...
PUM
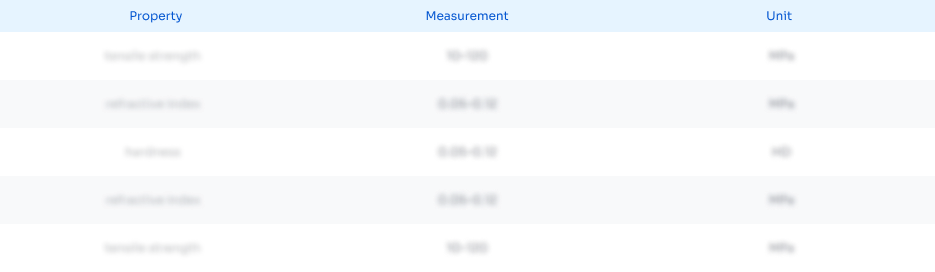
Abstract
Description
Claims
Application Information
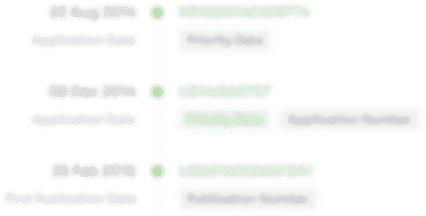
- R&D
- Intellectual Property
- Life Sciences
- Materials
- Tech Scout
- Unparalleled Data Quality
- Higher Quality Content
- 60% Fewer Hallucinations
Browse by: Latest US Patents, China's latest patents, Technical Efficacy Thesaurus, Application Domain, Technology Topic, Popular Technical Reports.
© 2025 PatSnap. All rights reserved.Legal|Privacy policy|Modern Slavery Act Transparency Statement|Sitemap|About US| Contact US: help@patsnap.com