Image segmentation method based on a secondary confinement region growth method
A technology for limiting areas and image segmentation, applied in image analysis, image data processing, instruments, etc., can solve problems such as being easily affected by noise, and achieve good results, easy control, and more complete areas
- Summary
- Abstract
- Description
- Claims
- Application Information
AI Technical Summary
Problems solved by technology
Method used
Image
Examples
Embodiment 1
[0117] Fig. 4 is a comparison diagram of common image segmentation effects, wherein Fig. 4(a) is the original image, and Fig. 4(b) is the result processed by the method of the present invention. In this embodiment, using the algorithm of the present invention, the number of seed points is set to 200 in step 2, the threshold is set to 30, the number of initial regions is 8 in step 5, the number of iterations is 30, and the predetermined value of energy change is 0. Fig. 4 (a) original picture is processed according to the method of the present invention, obtains the result image of Fig. 4 (b); As a comparison result, Fig. 4 (c) is the effect of the image segmentation algorithm based on the pixel point application hidden Markov model , the results are all obtained under the same clustering number, iteration times and energy change predetermined value settings, it can be seen that the pixel-based hidden Markov model divides objects of different categories such as planks and fruits...
Embodiment 2
[0119] In order to illustrate the segmentation effect of the method of the present invention when the image texture and details are relatively rich, the image in Figure 5(a) is used as the processing object for processing, which is implemented as this embodiment, and Figure 5(a) is without The original figure of processing, Fig. 5 (b) is the result figure after the process of the inventive method, in the application of the inventive method, in the step 2, the number of seed points is set to 200, and the threshold is set to 40, and in the step 5, the number of initial regions is 5, the number of iterations is 30, and the predetermined value of energy change is 0. Input figure (a) to get the result of figure (b). As a comparison result, Figure (c) is the effect of the image segmentation algorithm based on the application of the hidden Markov model based on the pixels of the original image. The results are all obtained under the same cluster number, iteration number and energy ch...
PUM
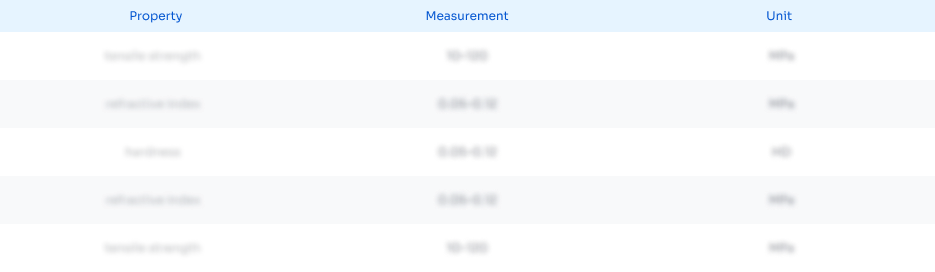
Abstract
Description
Claims
Application Information
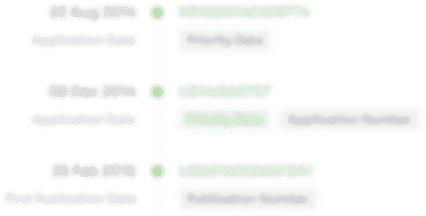
- R&D
- Intellectual Property
- Life Sciences
- Materials
- Tech Scout
- Unparalleled Data Quality
- Higher Quality Content
- 60% Fewer Hallucinations
Browse by: Latest US Patents, China's latest patents, Technical Efficacy Thesaurus, Application Domain, Technology Topic, Popular Technical Reports.
© 2025 PatSnap. All rights reserved.Legal|Privacy policy|Modern Slavery Act Transparency Statement|Sitemap|About US| Contact US: help@patsnap.com