electroencephalogram signal classification method based on a Gaussian Bernoulli convolution deep belief network
A technology of deep belief network and EEG signal, which is applied in the field of EEG classification signal based on Gaussian Bernoulli convolution deep belief network, can solve the problems of high fitting and calculation costs, reduce negative weights and reduce training time , the effect of improving the accuracy
- Summary
- Abstract
- Description
- Claims
- Application Information
AI Technical Summary
Problems solved by technology
Method used
Image
Examples
Embodiment Construction
[0033] The technical solutions in the embodiments of the present invention will be described clearly and in detail below with reference to the drawings in the embodiments of the present invention. The described embodiments are only some of the embodiments of the invention.
[0034] The technical scheme that the present invention solves the problems of the technologies described above is:
[0035] In independent component analysis filtering, the most critical step is the selection of independent components. The present invention adopts the BerlinIva data set to carry out the preprocessing experiment, and includes three clinical electrooculogram channels, which is convenient for researchers to carry out the research on the electrooculogram interference removal algorithm. Taking A01T as an example, the waveforms of each signal channel are as follows: figure 1 shown.
[0036] After independent component analysis and filtering, the EEG data waveforms of each channel are as follo...
PUM
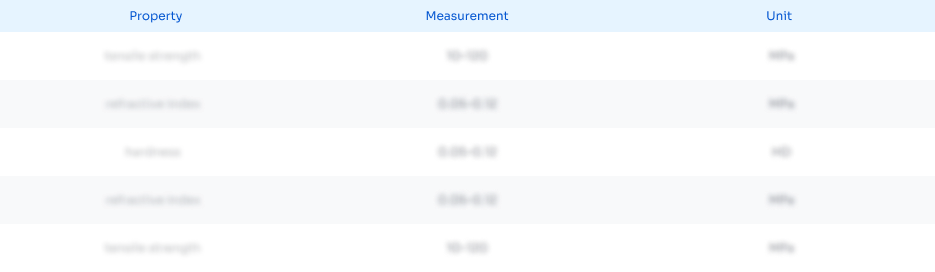
Abstract
Description
Claims
Application Information
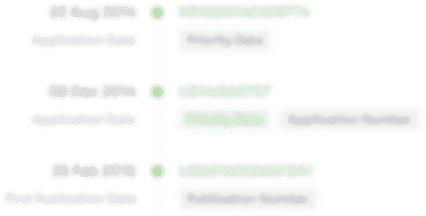
- R&D Engineer
- R&D Manager
- IP Professional
- Industry Leading Data Capabilities
- Powerful AI technology
- Patent DNA Extraction
Browse by: Latest US Patents, China's latest patents, Technical Efficacy Thesaurus, Application Domain, Technology Topic, Popular Technical Reports.
© 2024 PatSnap. All rights reserved.Legal|Privacy policy|Modern Slavery Act Transparency Statement|Sitemap|About US| Contact US: help@patsnap.com