Process industry system prediction module based on crossed relevance time-lag gray correlation analysis
A gray relational analysis and process industry technology, applied in the field of process industry production, can solve problems such as modeling errors, parameter perturbation noise and interference, and unsuitable multivariate problems
- Summary
- Abstract
- Description
- Claims
- Application Information
AI Technical Summary
Problems solved by technology
Method used
Image
Examples
Embodiment Construction
[0056] In the process industry, the prediction of key indicators can provide effective help for fault prediction and diagnosis analysis. After determining the indicators to be predicted and related indicators, the process industry system prediction model based on cross-correlation time-delay gray correlation analysis proposed by the present invention determines the delay time of each indicator variable and the indicators to be predicted on the basis of completing the elimination of data errors, and selects Appropriate index variables with a strong correlation with the to-be-predicted index are selected, and the delay time is combined with the artificial neural network prediction model to remove irrelevant and redundant index variables with a progressive selection strategy, optimize model parameters, and finally realize the to-be-predicted index effective prediction.
[0057] Such as figure 1 Shown, the specific implementation steps of the present invention include:
[0058] ...
PUM
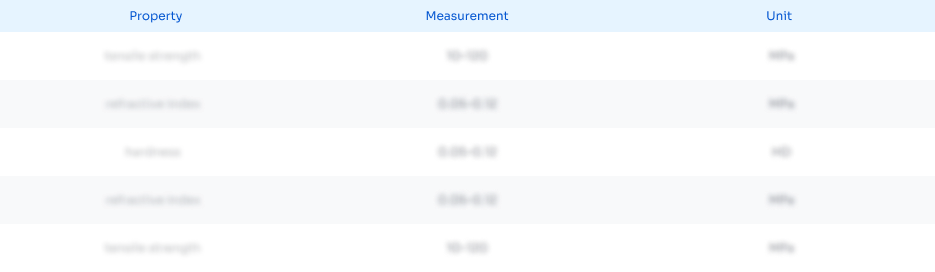
Abstract
Description
Claims
Application Information
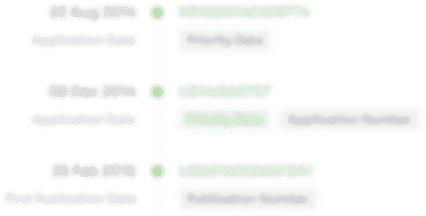
- R&D Engineer
- R&D Manager
- IP Professional
- Industry Leading Data Capabilities
- Powerful AI technology
- Patent DNA Extraction
Browse by: Latest US Patents, China's latest patents, Technical Efficacy Thesaurus, Application Domain, Technology Topic, Popular Technical Reports.
© 2024 PatSnap. All rights reserved.Legal|Privacy policy|Modern Slavery Act Transparency Statement|Sitemap|About US| Contact US: help@patsnap.com