New energy consumption method based on depth learning
A deep learning and new energy technology, applied in instruments, adaptive control, control/regulation systems, etc., can solve the problems of long distance of power transmission, difficult to accurately model, and the effect of conventional absorption algorithm is difficult to meet actual needs, etc. achieve universal adaptability
- Summary
- Abstract
- Description
- Claims
- Application Information
AI Technical Summary
Problems solved by technology
Method used
Image
Examples
Embodiment
[0157] The main purpose of this embodiment is to verify the effectiveness and rapidity of convergence of the algorithm of the present invention. In the experiment, a total of 17,376 groups of wind and solar power generation and consumption data in Gansu from January to June 2018 were used as the training set for training. The learning rate was uniformly set to 1.5, the adaptive update coefficient adjustment range was 0 to 2, and the number of training iterations was the largest. The value is 1000 and the training accuracy is 10 -3 . The training results of the algorithm of the present invention and the conventional dynamic recursive neural network are as follows: Figure 5 shown. It can be seen from the figure that the two algorithms can finally meet the convergence accuracy requirements, and the algorithm of the present invention has a faster convergence speed in the early stage of training, and the number of iterations required to achieve the training accuracy is less.
PUM
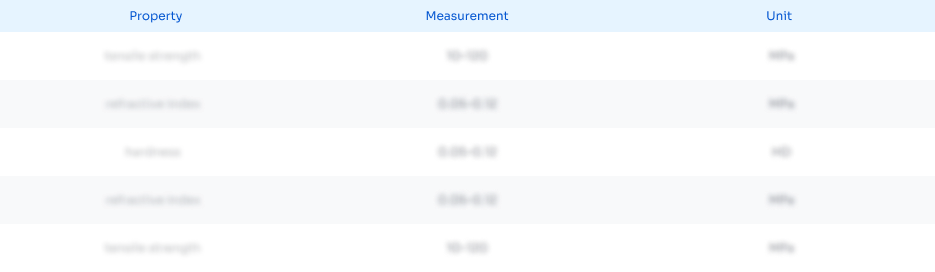
Abstract
Description
Claims
Application Information
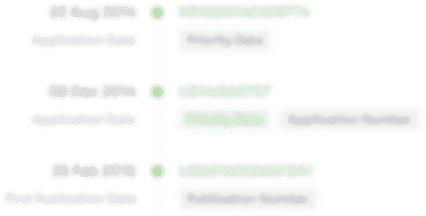
- R&D Engineer
- R&D Manager
- IP Professional
- Industry Leading Data Capabilities
- Powerful AI technology
- Patent DNA Extraction
Browse by: Latest US Patents, China's latest patents, Technical Efficacy Thesaurus, Application Domain, Technology Topic, Popular Technical Reports.
© 2024 PatSnap. All rights reserved.Legal|Privacy policy|Modern Slavery Act Transparency Statement|Sitemap|About US| Contact US: help@patsnap.com