Hyperspectral Image Classification Method Based on Superpixel Sample Expansion and Generative Adversarial Network
A technology of hyperspectral image and sample expansion, applied in the field of image classification, hyperspectral image classification based on superpixel expansion and generative confrontation network, can solve the problem of low classification accuracy, difficult determination of RGF execution times, and insufficient spectral-spatial information Combination and other issues to achieve the effect of improving accuracy, increasing the number of training samples, and enhancing feature extraction capabilities
- Summary
- Abstract
- Description
- Claims
- Application Information
AI Technical Summary
Problems solved by technology
Method used
Image
Examples
Embodiment Construction
[0042] The present invention will be described in further detail below in conjunction with the accompanying drawings and specific embodiments.
[0043] refer to figure 1 , realize the concrete steps of the present invention as follows:
[0044] Step 1, construct the initial training set and test set.
[0045] Commonly used hyperspectral image datasets include the Pavia University dataset obtained by NASA's ROSIS spectrometer, and the Indian Pines and Salinas datasets obtained by the airborne visible / infrared imaging spectrometer AVIRIS of NASA's Jet Propulsion Laboratory.
[0046] input hyperspectral image h p is a spectral vector, representing the vector formed by the reflection values of pixel p in each band, T is the total number of pixels in the hyperspectral image; the hyperspectral image H contains c-type pixels, of which M have labels Pixel, N unlabeled pixels, each pixel is a sample;
[0047] Use M labeled pixels as initial training samples to form an initial t...
PUM
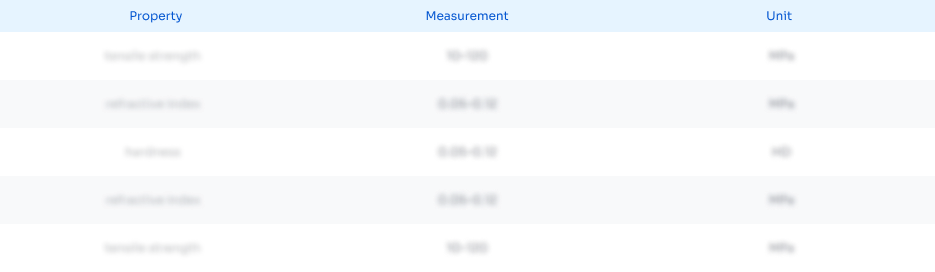
Abstract
Description
Claims
Application Information
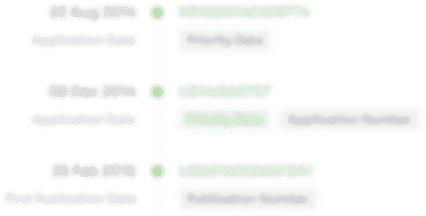
- R&D Engineer
- R&D Manager
- IP Professional
- Industry Leading Data Capabilities
- Powerful AI technology
- Patent DNA Extraction
Browse by: Latest US Patents, China's latest patents, Technical Efficacy Thesaurus, Application Domain, Technology Topic, Popular Technical Reports.
© 2024 PatSnap. All rights reserved.Legal|Privacy policy|Modern Slavery Act Transparency Statement|Sitemap|About US| Contact US: help@patsnap.com