Capsule network training method, classification method and system, equipment and storage medium
A technology of training method and classification method, which is applied in the direction of biological neural network model, text database clustering/classification, special data processing application, etc. It can solve multi-label text without providing iterative objective function, fixed number of iterations, capsule network training effect In order to solve problems such as poor classification effect, the number of iterations can be flexibly determined and the classification effect is better.
- Summary
- Abstract
- Description
- Claims
- Application Information
AI Technical Summary
Problems solved by technology
Method used
Image
Examples
Embodiment 1
[0039] In this embodiment, the capsule network includes such as figure 1 Structure shown:
[0040] The convolution layer 101 is used to extract feature scalar from the training sample text and construct the feature scalar group.
[0041] Specifically, the training sample text may be the text corresponding to the Wikipedia webpage, the text corresponding to the customer comments, the text corresponding to the biological gene sequence, or the text corresponding to the disease diagnosis certificate. Then, using the trained capsule network, it can finally be used for the label classification of the corresponding scene, such as: the classification of heterogeneous labels on Wikipedia web pages, the ratings of different aspects of customer reviews, gene function prediction in biology, disease prediction or diagnosis, etc. . Especially for large-scale multi-label text data sets, the amount of data is huge and the number of tags is tens of thousands. For example, the European Union Laws a...
Embodiment 2
[0058] Based on the first embodiment, this embodiment further provides the following content:
[0059] The training method of this embodiment also includes:
[0060] First, compress each initial vector to obtain a compact vector;
[0061] Secondly, the compact vector is subjected to affine transformation to obtain the intermediate vector.
[0062] In other words, as figure 2 As shown, in the capsule network, between the primary capsule layer 102 and the polymer capsule layer 103, a corresponding compact capsule layer 201 is also provided. The compact capsule layer 201 is used to perform the initial vector transfer from the primary capsule layer 102. The compact vector is obtained by compression processing, and after the compact vector reaches the polymerized capsule layer 103, the intermediate vector is obtained by the affine transformation of the polymerized capsule layer 103.
[0063] The compression processing may specifically include the following content: performing denoising pro...
Embodiment 3
[0066] On the basis of this embodiment and the first or second embodiment, the following content is further provided:
[0067] The training method of this embodiment also includes:
[0068] Under the condition of minimum classification loss, the transformation parameters corresponding to the affine transformation processing and the convolution parameters corresponding to the capsule network processing are updated iteratively.
[0069] Specifically, the routing parameters used in routing processing are updated iteratively through the above iterative routing mechanism. In the capsule network, other parameters, such as the transformation matrix of affine transformation, convolution parameters, etc., can all be based on preset classification loss targets. Under the condition of minimizing the function, the corresponding iterative update is performed to complete the training of the entire capsule network.
PUM
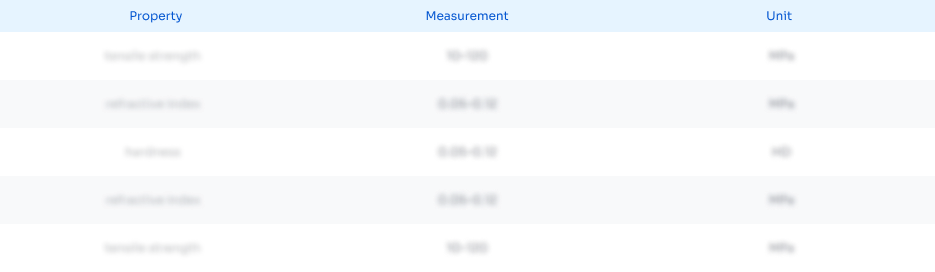
Abstract
Description
Claims
Application Information
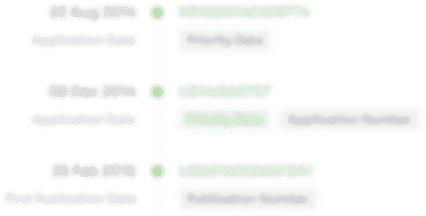
- Generate Ideas
- Intellectual Property
- Life Sciences
- Materials
- Tech Scout
- Unparalleled Data Quality
- Higher Quality Content
- 60% Fewer Hallucinations
Browse by: Latest US Patents, China's latest patents, Technical Efficacy Thesaurus, Application Domain, Technology Topic, Popular Technical Reports.
© 2025 PatSnap. All rights reserved.Legal|Privacy policy|Modern Slavery Act Transparency Statement|Sitemap|About US| Contact US: help@patsnap.com