Service providing system and method based on deep learning
A deep learning and service terminal technology, applied in the direction of neural learning methods, neural architecture, biological neural network models, etc., can solve problems such as lowering service quality, training data privacy leakage, and reducing the availability of compressed models, so as to improve service quality and protect Effects of Privacy, High Availability
- Summary
- Abstract
- Description
- Claims
- Application Information
AI Technical Summary
Problems solved by technology
Method used
Image
Examples
Embodiment
[0093] The method for generating a private compressed deep learning model of the deep learning-based service providing system provided by the embodiment of the present invention includes:
[0094] Step 1: Initialization.
[0095] Establish a convolutional neural network with two convolutional layers and three fully connected layers, and initialize the model parameters of the convolutional neural network Θ 0 . Define the input training data set X={x 1 ,x 2 ,...,x N}, the loss function L(Θ t ,X), Θ t Indicates the model parameters of the t-th round of iterative training. Define the constraint range G of the gradient, that is, the training sample x i The gradient value of is not greater than G, so the training sample x i The constrained gradient value is defined as where g t (x i ) represents the training sample x i Gradient during iterative training at round t.
[0096] Step 2: Privacy Dense Training.
[0097] Calculated according to the formula where c is a con...
PUM
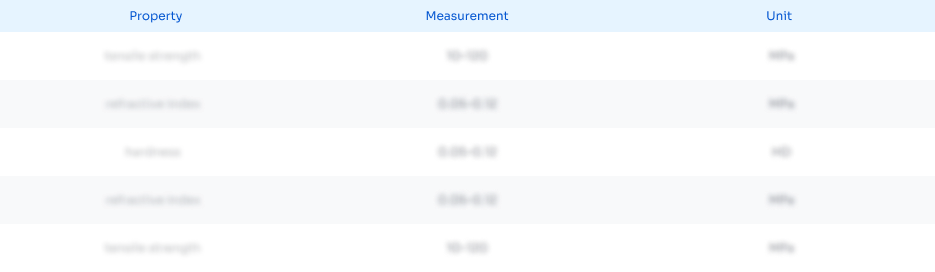
Abstract
Description
Claims
Application Information
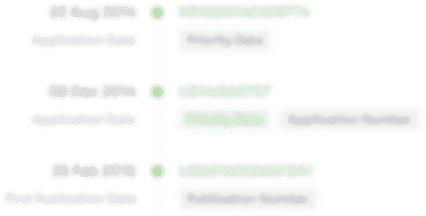
- R&D Engineer
- R&D Manager
- IP Professional
- Industry Leading Data Capabilities
- Powerful AI technology
- Patent DNA Extraction
Browse by: Latest US Patents, China's latest patents, Technical Efficacy Thesaurus, Application Domain, Technology Topic, Popular Technical Reports.
© 2024 PatSnap. All rights reserved.Legal|Privacy policy|Modern Slavery Act Transparency Statement|Sitemap|About US| Contact US: help@patsnap.com