A method of mm-wave sparse front channel estimation based on deep learning network
A deep learning network and channel estimation technology, which is applied in the field of millimeter wave sparse front channel estimation, can solve the problems of reducing estimation complexity, pilot overhead, channel estimation difficulties, etc., and achieves the effect of reducing complexity and reducing errors.
- Summary
- Abstract
- Description
- Claims
- Application Information
AI Technical Summary
Problems solved by technology
Method used
Image
Examples
Embodiment Construction
[0071]
[0072] Wherein, []
[0074]
[0080]
[0083]
[0084] Wherein, N is all possible numbers, C represents the formula of the number of combinations. N
[0086]
[0089]
[0090] where, (p∈{1,2,...,N
[0092]
[0093] where, (q∈{1,2,...,N
[0095]
[0096] Wherein, k∈{1,2,...,K} represents the kth subcarrier, and K represents the total number of subcarriers; represents the kth subcarrier
[0098] means rounded up.
[0100]Ω[k]=Φ[k]ΨP
[0103]
[0108] (2) Extract data from the database and divide it into two groups: training data and test data. Split the training data into complex values
[0110] (4) Construct two fully connected deep learning networks with the same network structure. Both are fully connected with two layers of forward feedback
[0111] The softmax function is defined as follows:
[0112]
[0114]
[0119]
[0122] The technical means disclosed by the solution of the present invention are not limited to the technical means disclosed in the above-mentioned ...
PUM
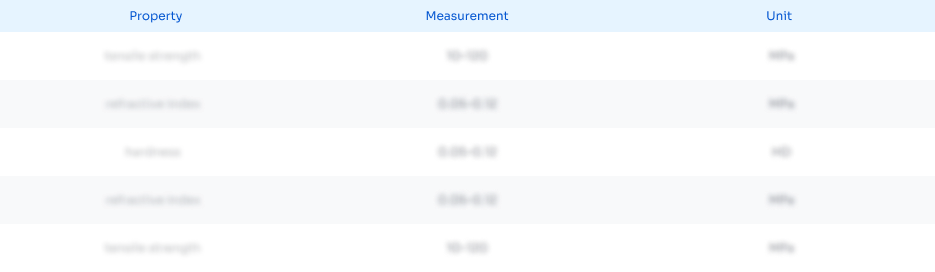
Abstract
Description
Claims
Application Information
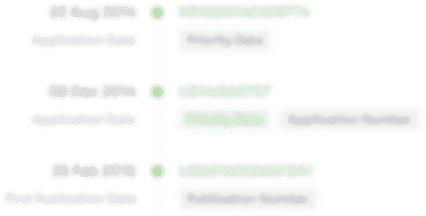
- R&D Engineer
- R&D Manager
- IP Professional
- Industry Leading Data Capabilities
- Powerful AI technology
- Patent DNA Extraction
Browse by: Latest US Patents, China's latest patents, Technical Efficacy Thesaurus, Application Domain, Technology Topic, Popular Technical Reports.
© 2024 PatSnap. All rights reserved.Legal|Privacy policy|Modern Slavery Act Transparency Statement|Sitemap|About US| Contact US: help@patsnap.com