Abnormal behavior detection method and system
A detection method and behavioral technology, applied in the field of intelligent identification, can solve the problems of missed detection and negative, cumbersome and inconvenient, and cannot meet the needs of video surveillance
- Summary
- Abstract
- Description
- Claims
- Application Information
AI Technical Summary
Problems solved by technology
Method used
Image
Examples
Embodiment 1
[0128] Such as figure 1 As shown, a single-person abnormal behavior detection method includes steps:
[0129] S1. Use the neural network human skeleton extraction model to extract the dynamic human skeleton joint points in the video to form a skeleton data set;
[0130] S2. Obtain a higher-level behavioral feature map corresponding to the skeleton through the ST-GCN (space-time graph convolution) network, that is, the surface behavioral feature;
[0131] S3. Input the behavior feature map into the abnormal behavior classifier model, and match to identify the behavior type;
[0132] S4. Use the yolov3 feature extraction model to detect dangerous goods, such as identifying and detecting knives;
[0133] 1, wherein, the establishment steps of described human skeleton extraction model are as follows:
[0134] Divide the human skeleton into five parts, namely two arms, two legs and a torso;
[0135] Use 3D conversion technology to select the physical structure of the joints and...
Embodiment 2
[0255] A multi-person abnormal behavior detection method, wherein multi-person pose estimation is based on single-person pose estimation, and the overall processing process of the model is:
[0256] ①Read a picture with width w×height h;
[0257] ②The 10-layer VGG-19 network is passed to train an image feature F that is also w×h;
[0258] ③Introduce two layers of different convolutional neural networks, you can get:
[0259] Key point confidence network S=(S 1 ,S 2 ,...,S J ) where J represents that there are J parts in the human body:
[0260] S j ∈R w×h ,j∈{1...J}.
[0261] Keypoint affinity vector field L c ∈R w×h×2 ,c∈{1,...,C}.
[0262] ④The key points are clustered to obtain the skeleton, and the schematic diagram of the key point confidence network and affinity vector field network is as follows: Figure 9 As shown, S is the confidence network, and L is the affinity vector field network:
[0263]
[0264]
[0265] The loss function of the whole model i...
PUM
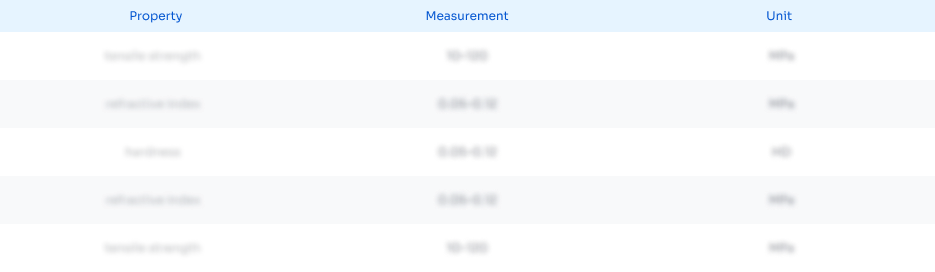
Abstract
Description
Claims
Application Information
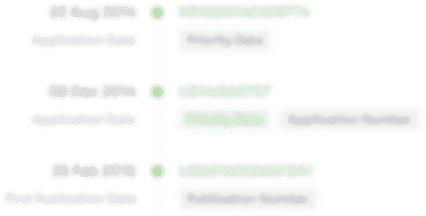
- R&D Engineer
- R&D Manager
- IP Professional
- Industry Leading Data Capabilities
- Powerful AI technology
- Patent DNA Extraction
Browse by: Latest US Patents, China's latest patents, Technical Efficacy Thesaurus, Application Domain, Technology Topic, Popular Technical Reports.
© 2024 PatSnap. All rights reserved.Legal|Privacy policy|Modern Slavery Act Transparency Statement|Sitemap|About US| Contact US: help@patsnap.com