Heart motion estimation method and system and terminal equipment
A motion estimation and heart technology, applied in the field of image processing, can solve problems such as difficult to determine the corresponding relationship of sampling points on the myocardial contour, difficult heart motion model estimation, etc., and achieve the effect of reducing the gradient disappearance phenomenon
- Summary
- Abstract
- Description
- Claims
- Application Information
AI Technical Summary
Problems solved by technology
Method used
Image
Examples
Embodiment 1
[0066] Such as figure 1 As shown, the embodiment of the present invention provides a heart motion estimation method, by constructing a left ventricle correspondence learning network, judging the left ventricle image feature pairs with corresponding relations, and using the deformation function to estimate the cardiac motion field according to these correspondence relations, effectively By overcoming the mismatch problem in the corresponding relationship, the corresponding relationship of the myocardium can be accurately extracted without segmenting the myocardium, thereby obtaining a stable and reasonable cardiac motion field. The heart motion estimation method includes but not limited to the following steps:
[0067] S101. Build a basic network model.
[0068] In the above step S101, the basic network model is a deep learning network with dense connections and atrous convolutions.
[0069] In specific applications, the deep learning network of densely connected mixed hole c...
Embodiment 2
[0108] Such as Figure 4 As shown, the embodiment of the present invention shows a schematic structural diagram of the basic network model of the left ventricle correspondence learning network in the first embodiment. Among them, the left ventricle correspondence learning network is based on the deep learning network of densely connected mixed hole convolution.
[0109] see Figure 4 , in the embodiment of the present invention, the basic network model 40 of the left ventricle correspondence learning network includes two densely connected networks with the same structure;
[0110] The densely connected network includes a two-layer cascaded traditional convolution network 41 (not shown in the figure) and a five-layer cascaded hole convolution network 42 (not shown in the figure);
[0111] Among them, the traditional convolutional network 41 and the hole convolutional network 42 are densely connected to each other;
[0112] Among them, the network parameters of the two-layer ...
Embodiment 3
[0124] The embodiment of the present invention is based on the heart motion estimation method provided in the first and second embodiments, and describes the construction and training of the left ventricle correspondence learning network, that is, step S101 to step S103 and their detailed implementation process, the implementation and implementation in practical applications. Realize the principle.
[0125] In the embodiment of the present invention, the assumed application scenario is cardiac motion estimation in which the image of the left ventricle is Cine MRI.
[0126] In the embodiment of the present invention, it starts from step S101, and step S101: build a basic network model. The implementation and implementation principle of step S101 in practical applications are as follows:
[0127] 1) Construct a deep learning network with densely connected mixed hole convolution.
[0128] Among them, the deep learning network of densely connected mixed hole convolution is compo...
PUM
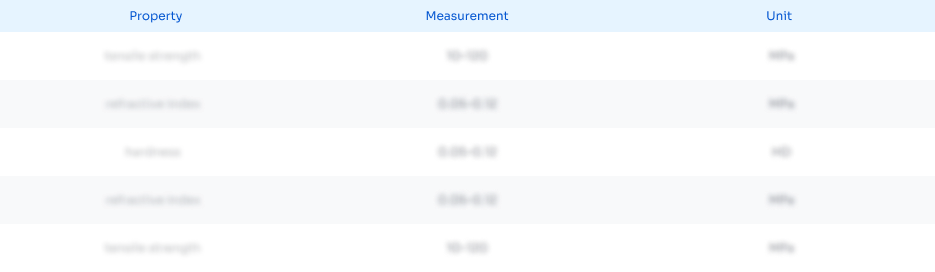
Abstract
Description
Claims
Application Information
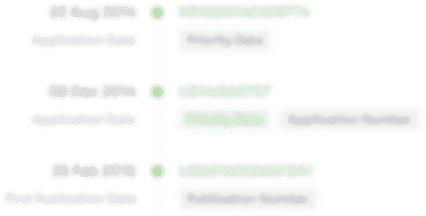
- R&D Engineer
- R&D Manager
- IP Professional
- Industry Leading Data Capabilities
- Powerful AI technology
- Patent DNA Extraction
Browse by: Latest US Patents, China's latest patents, Technical Efficacy Thesaurus, Application Domain, Technology Topic.
© 2024 PatSnap. All rights reserved.Legal|Privacy policy|Modern Slavery Act Transparency Statement|Sitemap