Speaker identification method based on joint optimization of total variation space and classifier
A technology of joint optimization and identification, applied in the field of speaker identification, can solve the problems of affecting system identification performance, high error rate of speaker identification, unfavorable identification tasks, etc.
- Summary
- Abstract
- Description
- Claims
- Application Information
AI Technical Summary
Problems solved by technology
Method used
Image
Examples
specific Embodiment approach 1
[0026] Specific implementation mode one: as figure 1 As shown, the speaker identification method based on the joint optimization of the total variation space and the classifier described in this embodiment comprises the following steps:
[0027] Step 1. Input the Mel cepstral coefficients of each segment of speech in the training set into the general background model, and use the maximum a posteriori probability method on the general background model to adapt to obtain the Gaussian mixture model corresponding to each segment of speech. Using the Gaussian mixture model Obtain the mean supervector corresponding to each segment of speech in the training set;
[0028] Then the mean supervector corresponding to each segment of speech in the training set forms the mean supervector set;
[0029] Step 2, calculate the covariance matrix Φ of the mean value m of the mean value supervector corresponding to all segments of speech in the training set and the corresponding mean value super...
specific Embodiment approach 2
[0044] Specific embodiment two: the difference between this embodiment and specific embodiment one is: the Gaussian mixture model is used to obtain the mean supervector corresponding to each section of speech in the training set, and its specific process is:
[0045] Suppose the training set contains a total of S 0 speaker's speech, and the total number of speech segments containing the sth speaker is H s , s=1,2,...,S 0 ;
[0046] According to the mean value μ of all Gaussian components corresponding to the h-th segment of speech of the s-th speaker c , c=1,2,...,C, obtain the mean supervector M corresponding to the h segment of the s speaker's speech s,h ,M s,h The expression is:
[0047]
[0048] Among them: C represents the number of mean values of the Gaussian components corresponding to the h-th segment of the s-th speaker’s speech, μ 1 Represents the mean value of the first Gaussian component corresponding to the h-th segment of speech of the s-th speaker;
...
specific Embodiment approach 3
[0050] Specific implementation mode three: the difference between this implementation mode and specific implementation mode two is: the specific process of said step two is:
[0051]
PUM
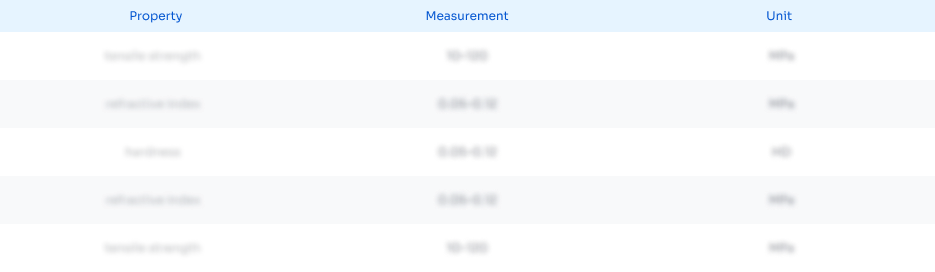
Abstract
Description
Claims
Application Information
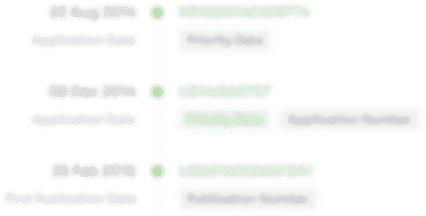
- R&D
- Intellectual Property
- Life Sciences
- Materials
- Tech Scout
- Unparalleled Data Quality
- Higher Quality Content
- 60% Fewer Hallucinations
Browse by: Latest US Patents, China's latest patents, Technical Efficacy Thesaurus, Application Domain, Technology Topic, Popular Technical Reports.
© 2025 PatSnap. All rights reserved.Legal|Privacy policy|Modern Slavery Act Transparency Statement|Sitemap|About US| Contact US: help@patsnap.com