Zero sample learning method and system based on semantic attribute attention redistribution mechanism
A technology of semantic attribute and sample learning, applied in computer parts, instruments, biological neural network models, etc., can solve problems such as misclassification, and achieve the effect of improving stability
- Summary
- Abstract
- Description
- Claims
- Application Information
AI Technical Summary
Problems solved by technology
Method used
Image
Examples
Embodiment Construction
[0069] The present invention will be further described in detail below with reference to the accompanying drawings and embodiments. It should be noted that the following embodiments are intended to facilitate the understanding of the present invention, but do not limit it in any way.
[0070] Such as figure 1 As shown, the main model of the present invention is divided into a visual feature module and three branch modules respectively corresponding to three outputs of the image input, and these three branches are synchronously put into the optimization process of the whole model. Specific steps are as follows:
[0071] (a) The visual feature module learns the input image x during the zero-shot learning training process i The deep visual features θ i , the basic steps are as follows:
[0072] (a-1) Use the pre-trained large-scale neural network ResNet101 to initialize the network model parameters. input image x i First, the center is cropped to an image x' of size 224×224 ...
PUM
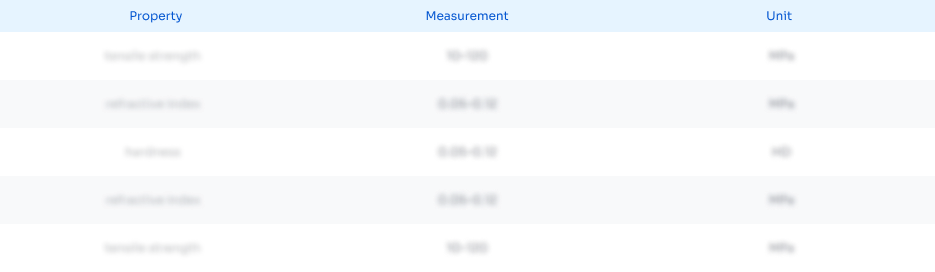
Abstract
Description
Claims
Application Information
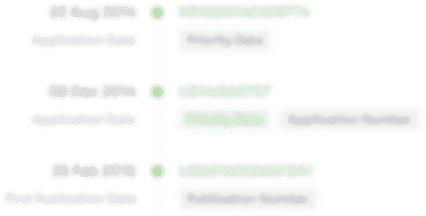
- R&D Engineer
- R&D Manager
- IP Professional
- Industry Leading Data Capabilities
- Powerful AI technology
- Patent DNA Extraction
Browse by: Latest US Patents, China's latest patents, Technical Efficacy Thesaurus, Application Domain, Technology Topic, Popular Technical Reports.
© 2024 PatSnap. All rights reserved.Legal|Privacy policy|Modern Slavery Act Transparency Statement|Sitemap|About US| Contact US: help@patsnap.com