Target counting method and system based on double-attention multi-scale cascade network
A multi-scale cascading and target counting technology, which is applied in the field of image processing, can solve problems such as counting deviation, achieve the effect of improving robustness and improving target counting deviation
- Summary
- Abstract
- Description
- Claims
- Application Information
AI Technical Summary
Problems solved by technology
Method used
Image
Examples
Embodiment 1
[0077] figure 1 It is a flow chart of the object counting method based on the dual-attention multi-scale cascaded network of the present invention. Such as figure 1 As shown, an object counting method based on dual-attention multi-scale cascade network, including:
[0078] Step 101: Acquire a grayscale-processed image.
[0079] Step 102: Input the grayscaled image to the initial module of the double-attention multi-scale cascade network for initial feature extraction to obtain an initial feature map.
[0080] The inception module consists of two convolutional layers. Input the grayscaled image into the first convolutional layer in the cascaded network to obtain a first initial feature map; input the first initial feature map into the second convolutional layer in the cascaded network layer to get the initial feature map. The convolution kernel sizes of the first convolution layer and the second convolution layer are both 9×9. The image generates 16 feature maps through t...
Embodiment 2
[0116] In addition to the steps described in Embodiment 1, Embodiment 2 of the present invention also includes after step 108:
[0117] Get loss functions for fully connected layers and feature extraction operations.
[0118] Weighting the loss function of the fully connected layer and the feature extraction operation to obtain the overall loss function of the cascaded network; the loss function of the fully connected layer adopts a cross entropy function, and the loss function of the feature extraction operation is an estimated target The Euclidean distance between the distribution density map and the true target distribution density map.
[0119] An error of the cascaded network is determined from an overall loss function of the cascaded network.
[0120] The error is backpropagated, the weight parameters of the cascaded network are updated, and a trained model for object counting is obtained through multiple iterations.
Embodiment 3
[0122] An object counting system based on a dual-attention multi-scale cascaded network, including:
[0123] The acquisition module is used to acquire the grayscale processed image.
[0124] The first feature extraction module is used to input the image after the grayscale processing to the initial module of the double-attention multi-scale cascade network for initial feature extraction to obtain an initial feature map; the initial module includes two convolutions layer.
[0125] The low-level detail feature map and the high-level semantic feature map determination module are used to input the initial feature map to the first branch network of the double-attention multi-scale cascaded network to obtain the low-level detail feature map and the high-level semantic feature map; The first branch network includes multiple convolutional layers and multiple pooling layers.
[0126] A transformation module, configured to perform channel attention transformation on the low-level deta...
PUM
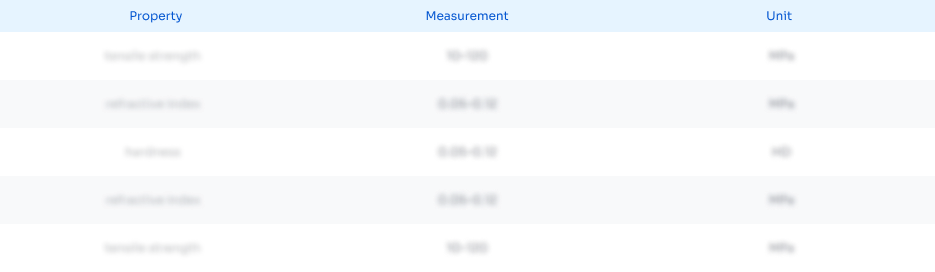
Abstract
Description
Claims
Application Information
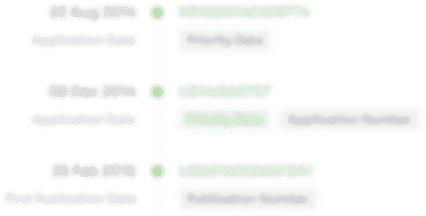
- Generate Ideas
- Intellectual Property
- Life Sciences
- Materials
- Tech Scout
- Unparalleled Data Quality
- Higher Quality Content
- 60% Fewer Hallucinations
Browse by: Latest US Patents, China's latest patents, Technical Efficacy Thesaurus, Application Domain, Technology Topic, Popular Technical Reports.
© 2025 PatSnap. All rights reserved.Legal|Privacy policy|Modern Slavery Act Transparency Statement|Sitemap|About US| Contact US: help@patsnap.com