Method and device for serialized feature extraction and classification of corneal disease images based on deep neural network
A deep neural network and feature extraction technology, which is applied in the field of corneal disease image classification, can solve problems such as neglect, difficulty in obtaining classification accuracy, and inability to provide reasonable explanations for classification results, achieving superior performance and high-level diagnostic results
- Summary
- Abstract
- Description
- Claims
- Application Information
AI Technical Summary
Problems solved by technology
Method used
Image
Examples
Embodiment
[0093] This embodiment is tested on the corneal disease image data set provided by the Department of Ophthalmology, Run Run Shaw Hospital Affiliated to Zhejiang University School of Medicine. This method mainly classifies and recognizes the three corneal diseases with the highest incidence rate and the greatest recognition value: bacterial keratitis, fungal keratitis and viral keratitis, and other corneal diseases that are not the above three categories are classified into one category, so The algorithm identified each corneal disease image as one of four categories: bacterial keratitis, fungal keratitis, viral keratitis, and other corneal disease.
[0094] In the algorithm training and testing, the relevant data of 867 patients with corneal diseases were sorted out. The data corresponding to each patient includes personal basic information, disease etiology basis, diagnosis conclusion, several slit lamp photographed images and lesion area mask annotations, and structured chie...
PUM
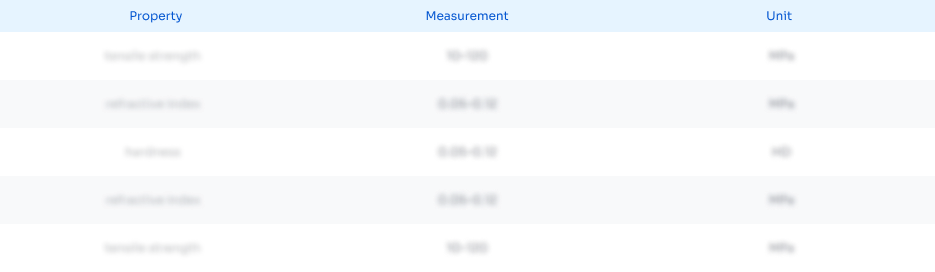
Abstract
Description
Claims
Application Information
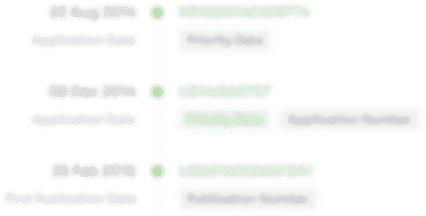
- R&D Engineer
- R&D Manager
- IP Professional
- Industry Leading Data Capabilities
- Powerful AI technology
- Patent DNA Extraction
Browse by: Latest US Patents, China's latest patents, Technical Efficacy Thesaurus, Application Domain, Technology Topic, Popular Technical Reports.
© 2024 PatSnap. All rights reserved.Legal|Privacy policy|Modern Slavery Act Transparency Statement|Sitemap|About US| Contact US: help@patsnap.com