Scratch image data amplification method based on generative adversarial network
A technology of image data and image data sets, which is applied in the field of computer vision, can solve the problems of small number of surface defect images, time-consuming, difficult collection and labeling, etc., and achieve the effect of reducing the statistical law difference of probability distribution, meeting the quantity demand, and reducing the demand
- Summary
- Abstract
- Description
- Claims
- Application Information
AI Technical Summary
Problems solved by technology
Method used
Image
Examples
Embodiment 1
[0042] Such as figure 1 As shown, this embodiment provides a method for augmenting scratch image data based on a generative confrontation network, including the following steps:
[0043] Step S1, constructing a scratch image data set;
[0044] Step S2, scratch image preprocessing;
[0045] Step S3, constructing a generative confrontation network;
[0046] Step S4, confrontation training;
[0047] Step S5, outputting a scratch image augmentation data set.
[0048] Specifically, in step S1, constructing a scratch image data set includes the following steps:
[0049] Step S1.1, collect the surface image of the product through the CCD camera, and select the image with scratches as the training image, such as Figure 2a-Figure 2c As shown, the rest of the scratch-free images are used as background images for subsequent composite images;
[0050] Step S1.2, manually mark the scratch position, and perform binarization processing on the marked image, such as Figure 3a-Figure 3...
PUM
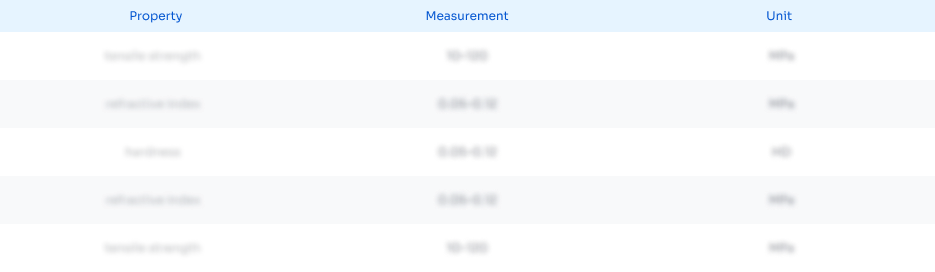
Abstract
Description
Claims
Application Information
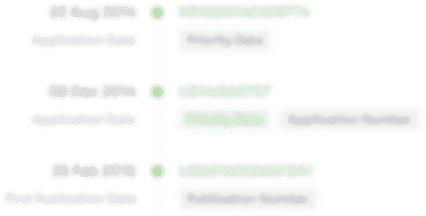
- R&D Engineer
- R&D Manager
- IP Professional
- Industry Leading Data Capabilities
- Powerful AI technology
- Patent DNA Extraction
Browse by: Latest US Patents, China's latest patents, Technical Efficacy Thesaurus, Application Domain, Technology Topic, Popular Technical Reports.
© 2024 PatSnap. All rights reserved.Legal|Privacy policy|Modern Slavery Act Transparency Statement|Sitemap|About US| Contact US: help@patsnap.com