Model generation method and system, semantic recognition method and system, equipment and storage medium
A technology of model generation and semantic recognition, applied in the field of semantic recognition, can solve the problems of inflexibility and high labor costs, and achieve the effect of improving accuracy, user experience and click conversion rate
- Summary
- Abstract
- Description
- Claims
- Application Information
AI Technical Summary
Problems solved by technology
Method used
Image
Examples
Embodiment 1
[0074] Such as figure 1 As shown, the method for model generation provided in this embodiment includes the following steps:
[0075] Step 101, acquiring historical data.
[0076] Step 102, perform word segmentation and labeling on each piece of historical data to obtain a corresponding first historical sequence.
[0077] In step 102 of this embodiment, each of the first history sequences includes words after word segmentation and tagged tags corresponding to each word after word segmentation; the tags include the central word of the item and the modifiers of the item , inquiry range, brand and channel number of the item, each of the tags also includes position information of the corresponding word after word segmentation in the corresponding historical data, and the position information includes a start position, an intermediate position and / or or end position. In this embodiment, the tag adopts One-Hot (one-hot code) encoding.
[0078] Step 103, using CNN to perform chara...
Embodiment 2
[0093] Such as figure 2 As shown, the system of model generation of this embodiment includes:
[0094] The first acquisition module 1 is used to acquire historical data.
[0095] The first processing module 2 is used to segment and label each piece of historical data to obtain a corresponding first historical sequence, and each of the first historical sequences includes word after word segmentation and each word after word segmentation The corresponding label after the annotation.
[0096] The second processing module 3 is configured to use CNN to perform character-level feature extraction on each of the first historical sequences to obtain a second historical sequence, and the second historical sequence includes the information of the first historical sequence and the corresponding The extracted character-level features.
[0097] The third processing module 4 is used to use bidirectional LSTM to perform word-level feature extraction on each of the second historical sequen...
Embodiment 3
[0107] image 3 A schematic structural diagram of a model generation device provided by Embodiment 3 of the present invention. image 3 A block diagram of an exemplary model generation apparatus 30 suitable for implementing embodiments of the invention is shown. image 3 The shown model generating device 30 is only an example, and should not impose any limitation on the functions and scope of use of the embodiments of the present invention.
[0108] Such as image 3 As shown, the model-generating device 30 may take the form of a general-purpose computing device, which may be a server device, for example. Components of the model generation device 30 may include but not limited to: the at least one processor 31 , the at least one memory 32 , and the bus 33 connecting different system components (including the memory 32 and the processor 31 ).
[0109] The bus 33 includes a data bus, an address bus, and a control bus.
[0110] The memory 32 may include a volatile memory, such...
PUM
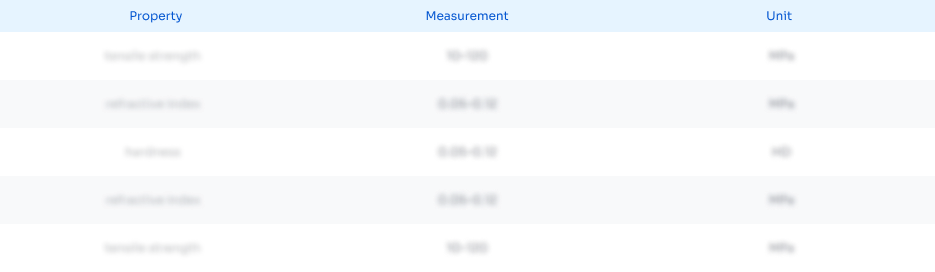
Abstract
Description
Claims
Application Information
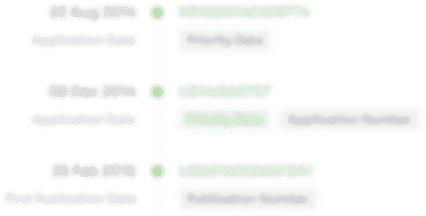
- R&D Engineer
- R&D Manager
- IP Professional
- Industry Leading Data Capabilities
- Powerful AI technology
- Patent DNA Extraction
Browse by: Latest US Patents, China's latest patents, Technical Efficacy Thesaurus, Application Domain, Technology Topic, Popular Technical Reports.
© 2024 PatSnap. All rights reserved.Legal|Privacy policy|Modern Slavery Act Transparency Statement|Sitemap|About US| Contact US: help@patsnap.com