CycleGAN-based image training network structure ArcGAN and method
A technology of network structure and network architecture, applied in the field of deep learning and image processing, can solve problems such as poor application of deep network structure, style migration of color architectural drawings, inability to achieve stylization, etc., to achieve excellent visual effects, The effect of rich and flexible colors and improved feature utilization
- Summary
- Abstract
- Description
- Claims
- Application Information
AI Technical Summary
Problems solved by technology
Method used
Image
Examples
Embodiment Construction
[0041] The present invention will be described in further detail below in conjunction with the accompanying drawings and specific embodiments. It should be understood that the specific embodiments described here are only used to explain the present invention, not to limit the present invention.
[0042] The CycleGAN network architecture consists of two convolutional neural networks (CNNs), a generator G that is trained to produce outputs that fool the discriminator. The other is the discriminator D, which classifies whether an image is from a real object or a synthetic image. In order to adapt to the particularity of the building model, the network structure ArcGAN of the present invention is mainly composed of a generator and a double discriminator, and the double discriminator includes a rough discriminator (CD) and a fine discriminator (FD). For details, see figure 1 .
[0043] 1. About the generator
[0044] The generator is mainly responsible for generating a picture ...
PUM
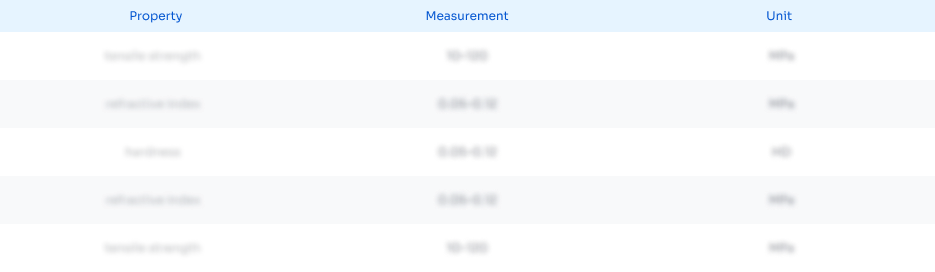
Abstract
Description
Claims
Application Information
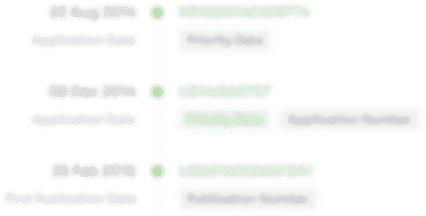
- R&D Engineer
- R&D Manager
- IP Professional
- Industry Leading Data Capabilities
- Powerful AI technology
- Patent DNA Extraction
Browse by: Latest US Patents, China's latest patents, Technical Efficacy Thesaurus, Application Domain, Technology Topic, Popular Technical Reports.
© 2024 PatSnap. All rights reserved.Legal|Privacy policy|Modern Slavery Act Transparency Statement|Sitemap|About US| Contact US: help@patsnap.com