Scalp EEG feature extraction and classification method based on end-to-end convolutional neural network
A technology of convolutional neural network and classification method, which is applied in the field of scalp EEG feature extraction and classification based on end-to-end convolutional neural network, which can solve the problems of limited data volume, time-consuming and labor-intensive, complex parameter volume of deep neural network, etc. , to achieve high classification accuracy, simple structure, and enhanced network robustness
- Summary
- Abstract
- Description
- Claims
- Application Information
AI Technical Summary
Problems solved by technology
Method used
Image
Examples
Embodiment
[0036] Such as figure 2 As shown, the scalp EEG feature extraction and classification method based on the end-to-end convolutional neural network collects the original scalp EEG signals as training data in the training network stage, firstly performs data enhancement on the collected training data, and then lets the enhanced training The data is filtered by three band-pass filters, and finally the convolutional neural network is trained with the filtered training data; specifically, the convolutional neural network is trained using the BP algorithm;
[0037] In the data detection stage, the collected data to be detected are input into the trained convolutional neural network for feature extraction and classification, including the following steps:
[0038] S1. Use the x raw Represented, and then filtered by three band-pass filters, the obtained signals are respectively represented by x θ 、x μ and x β express;
[0039] S2. For the filtered scalp EEG signal x θ 、x μ and ...
PUM
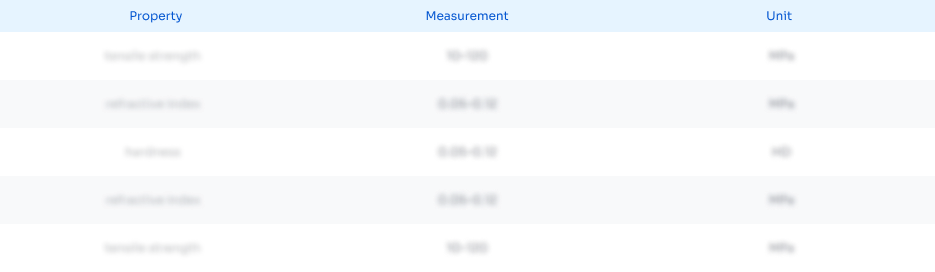
Abstract
Description
Claims
Application Information
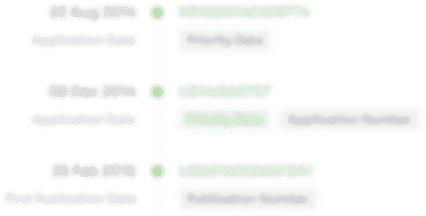
- R&D
- Intellectual Property
- Life Sciences
- Materials
- Tech Scout
- Unparalleled Data Quality
- Higher Quality Content
- 60% Fewer Hallucinations
Browse by: Latest US Patents, China's latest patents, Technical Efficacy Thesaurus, Application Domain, Technology Topic, Popular Technical Reports.
© 2025 PatSnap. All rights reserved.Legal|Privacy policy|Modern Slavery Act Transparency Statement|Sitemap|About US| Contact US: help@patsnap.com