A method for rapid prediction of the yellowing time of Yuanan yellow tea using artificial neural network with polynomial net structure
An artificial neural network and yellowing technology, applied in neural learning methods, biological neural network models, neural architecture, etc., can solve problems such as the inability to accurately grasp the yellowing time in real time and the quality of tea, and accurately predict the yellowing time of samples. , Objective prediction, the effect of reducing the amount of calculation
- Summary
- Abstract
- Description
- Claims
- Application Information
AI Technical Summary
Problems solved by technology
Method used
Image
Examples
specific Embodiment 1
[0082] (1) Collection and classification of fresh leaf samples
[0083] Collection of Anji white tea varieties from Yuan'an County, Hubei Province with single bud (sessile), one bud and one leaf (consisting of single bud, first leaf and longer stem) and one bud and two leaves (consisting of single bud, first leaf and second leaf) 120 fresh leaf samples from three different parts. After the sample is completed, the yellowing is carried out, and the yellowing time is accurately recorded at the same time. According to the different yellowing time, the samples were divided into calibration set and validation set according to the ratio of 3:1, of which 90 samples were in the calibration set and 30 samples were in the validation set, which were used to test the robustness of the calibration set model.
[0084] (2) Spectral scanning
[0085] The near-infrared spectra of all dull yellow samples were obtained by scanning with the Thermo Fisher Scientific Antaris II Fourier transform ...
PUM
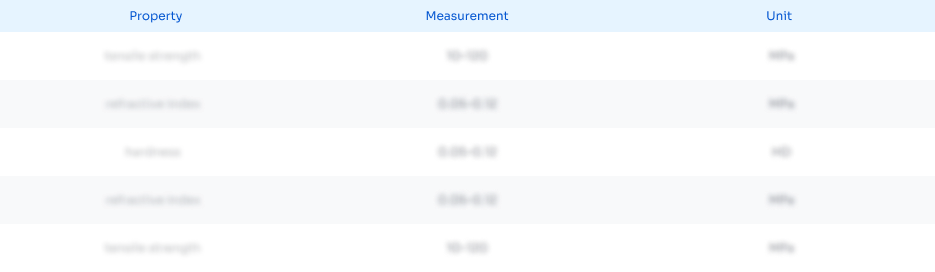
Abstract
Description
Claims
Application Information
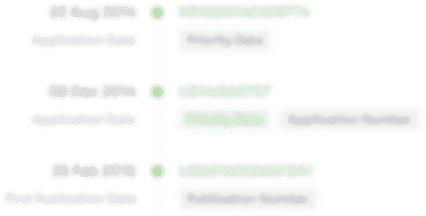
- R&D Engineer
- R&D Manager
- IP Professional
- Industry Leading Data Capabilities
- Powerful AI technology
- Patent DNA Extraction
Browse by: Latest US Patents, China's latest patents, Technical Efficacy Thesaurus, Application Domain, Technology Topic, Popular Technical Reports.
© 2024 PatSnap. All rights reserved.Legal|Privacy policy|Modern Slavery Act Transparency Statement|Sitemap|About US| Contact US: help@patsnap.com