Time sequence variable-length die body mining method
A motif mining and time series technology, applied in the field of information processing, can solve problems such as poor scalability and slow algorithm speed, and achieve the effects of high accuracy, cost reduction, and speed improvement
- Summary
- Abstract
- Description
- Claims
- Application Information
AI Technical Summary
Problems solved by technology
Method used
Image
Examples
Embodiment
[0069] In order to verify the effect of the present invention, the experiment uses part of the data set in the UCR as the experimental data, and the experiment will be carried out from two aspects, (1) for the detailed analysis of the data set, the results produced according to the steps in the specific embodiment; (2) with Compared with existing algorithms, the time performance and recognition accuracy of the algorithm of the present invention are analyzed.
[0070] The accuracy and scalability of FMPVLMD are analyzed separately based on the two data sets.
[0071] 1) Accuracy analysis, comparing FMVLMD with the MN method in [51] and the original VLMD algorithm. Accuracy-on-Detection (AoD) is used to calculate the overlap ratio between the phantom output by the algorithm and the phantom implanted to measure the accuracy of each algorithm.
[0072] 2) Based on the Dataset1 and Dataset2 datasets, compare the FMPVLMD method with the MPVLMD method without any acceleration strate...
PUM
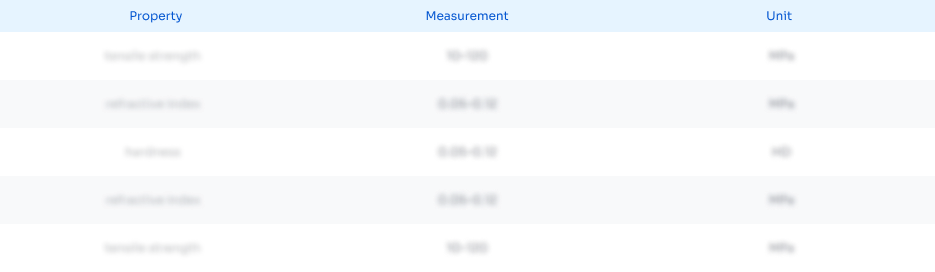
Abstract
Description
Claims
Application Information
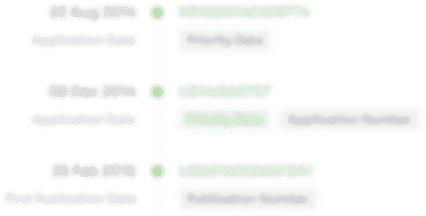
- R&D Engineer
- R&D Manager
- IP Professional
- Industry Leading Data Capabilities
- Powerful AI technology
- Patent DNA Extraction
Browse by: Latest US Patents, China's latest patents, Technical Efficacy Thesaurus, Application Domain, Technology Topic, Popular Technical Reports.
© 2024 PatSnap. All rights reserved.Legal|Privacy policy|Modern Slavery Act Transparency Statement|Sitemap|About US| Contact US: help@patsnap.com