Motor imagery electroencephalogram signal classification method of semi-supervised learning optimization SVM
A semi-supervised learning and motor imagery technology, applied in the computer field, can solve problems such as poor effect and difficult classification of motor imagery EEG signals, and achieve good classification results
- Summary
- Abstract
- Description
- Claims
- Application Information
AI Technical Summary
Problems solved by technology
Method used
Image
Examples
Embodiment 1
[0047] The present embodiment proposes the motor imagery EEG signal classification method of semi-supervised learning optimization SVM, such as figure 1 As shown, the method includes: obtaining motor imagery EEG signals --- motor imagery EEG preprocessing (step A) --- initialization (step B) --- self-training process (step C) --- imperialist competition algorithm Optimize parameters (step D) --- get classification rate on independent test set (step E)
[0048] The specific process is as follows:
[0049] Obtaining motor imagery EEG signals: In this embodiment, the data set IVa of the 2005 BCI competition is used to verify the effectiveness of the motor imagery EEG classification method using semi-supervised learning to optimize support vector machine parameters. In the experiment, 118 Ag / AgCl electrodes and a sampling frequency of 1000 Hz were used to record EEG, and a band-pass filter of 0.05 to 200 Hz was performed. Five subjects performed three motor imagery tasks in the ...
Embodiment 2
[0095] This embodiment adopts the semi-supervised imperial colonial competition algorithm optimization SVM (SSL-CCA-SVM, namely the method proposed in the above-mentioned embodiment 1), the supervised imperial colonial competitive algorithm optimization SVM (SL-CCA-SVM), and the semi-supervised particle swarm optimization algorithm Optimized SVM (SSL-PSO-SVM), supervised particle swarm optimization algorithm optimized SVM (SL-PSO-SVM), semi-supervised algorithm optimized standard SVM (SSL-SVM), and supervised algorithm optimized standard SVM (SL-SVM) were tested separately . The objective function of the particle swarm optimization algorithm is consistent with the imperial colonial competition algorithm.
[0096] For semi-supervised optimization SVM algorithm (SSL-CCA-SVM, SSL-PSO-SVM, SSL-SVM), supervised optimization SVM algorithm (SL-CCA-SVM, SL-PSO-SVM, SL-SVM), classification set samples In the process of 6*5-fold cross-validation, 176 (40 labeled samples, 136 unlabeled ...
PUM
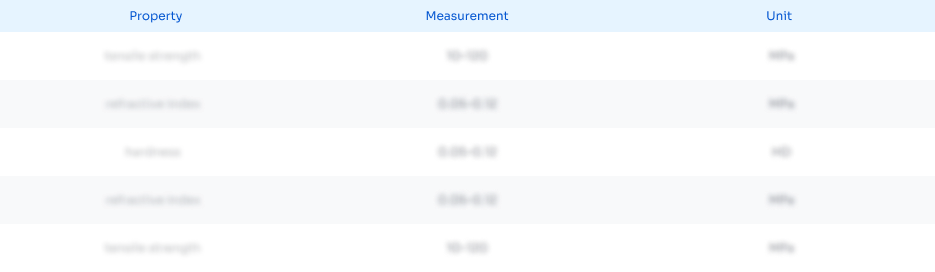
Abstract
Description
Claims
Application Information
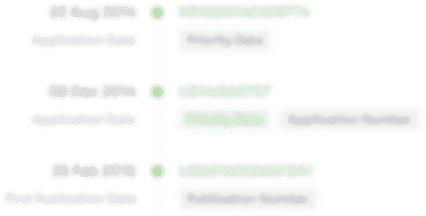
- R&D Engineer
- R&D Manager
- IP Professional
- Industry Leading Data Capabilities
- Powerful AI technology
- Patent DNA Extraction
Browse by: Latest US Patents, China's latest patents, Technical Efficacy Thesaurus, Application Domain, Technology Topic.
© 2024 PatSnap. All rights reserved.Legal|Privacy policy|Modern Slavery Act Transparency Statement|Sitemap