Real-time and accurate soft tissue deformation prediction
A technology of soft tissue and time, applied in medical data mining, diagnostic recording/measurement, image data processing, etc., can solve unsolved problems such as biomechanical models
- Summary
- Abstract
- Description
- Claims
- Application Information
AI Technical Summary
Problems solved by technology
Method used
Image
Examples
Embodiment Construction
[0016] Solvers based on biomechanics or other physics are constrained by the Courant-Friedrichs-Lewy condition in the size of the time increments used to solve the relevant partial differential equations. For animation, gaming, and / or medical imaging, this can lead to non-real-time modeling, thus hindering their use in these industries. To overcome this problem, a machine-learned artificial neural network is used to predict the output of a physics-based model at any time step that includes a larger than Courant-Friedrichs-Lewy condition for the solver increment. This may allow real-time and / or faster tissue deformation calculations in animation, gaming and / or medical imaging. Medical imaging is used in the example below. The same method or embodiment can be applied to tissue deformation in animation, games or other computer-based soft tissue modeling without loss of generality.
[0017] Real-time determination of soft tissue (bio)physics using learned deep neural networks. ...
PUM
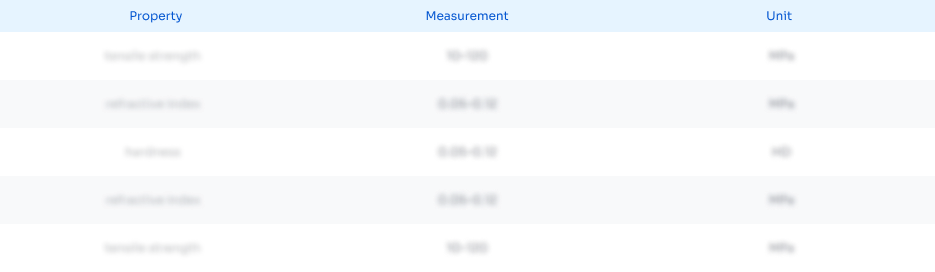
Abstract
Description
Claims
Application Information
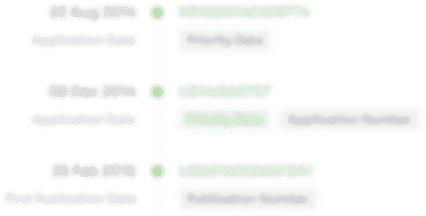
- R&D Engineer
- R&D Manager
- IP Professional
- Industry Leading Data Capabilities
- Powerful AI technology
- Patent DNA Extraction
Browse by: Latest US Patents, China's latest patents, Technical Efficacy Thesaurus, Application Domain, Technology Topic, Popular Technical Reports.
© 2024 PatSnap. All rights reserved.Legal|Privacy policy|Modern Slavery Act Transparency Statement|Sitemap|About US| Contact US: help@patsnap.com